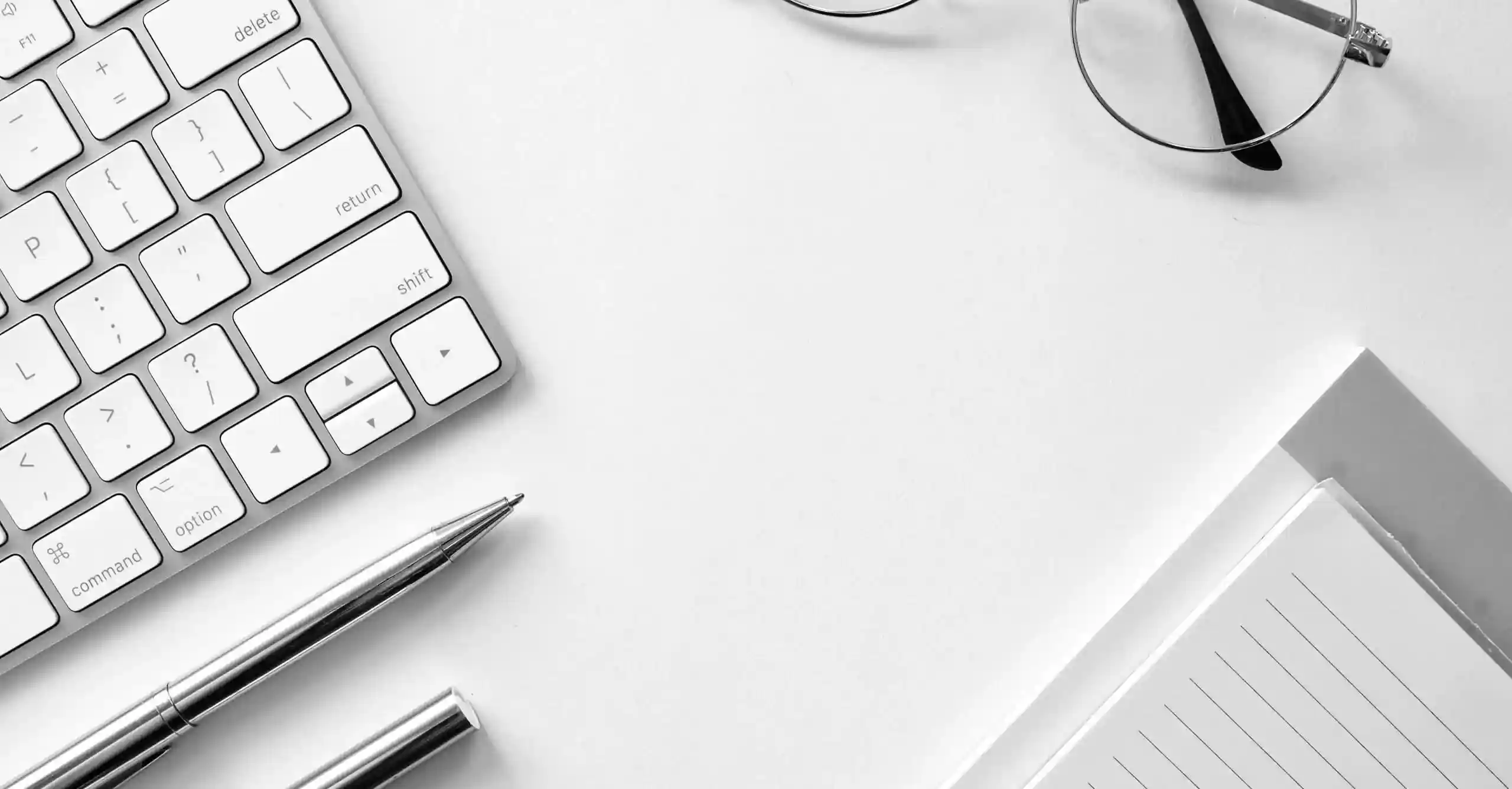
Blog, Data analysis has come of age
Sorry, nothing was found.
Sorry, nothing was found.
Cookie | Description |
---|---|
cli_user_preference | The cookie is set by the GDPR Cookie Consent plugin and is used to store the yes/no selection the consent given for cookie usage. It does not store any personal data. |
cookielawinfo-checkbox-advertisement | Set by the GDPR Cookie Consent plugin, this cookie is used to record the user consent for the cookies in the "Advertisement" category . |
cookielawinfo-checkbox-analytics | Set by the GDPR Cookie Consent plugin, this cookie is used to record the user consent for the cookies in the "Analytics" category . |
cookielawinfo-checkbox-necessary | This cookie is set by GDPR Cookie Consent plugin. The cookies is used to store the user consent for the cookies in the category "Necessary". |
CookieLawInfoConsent | The cookie is set by the GDPR Cookie Consent plugin and is used to store the summary of the consent given for cookie usage. It does not store any personal data. |
viewed_cookie_policy | The cookie is set by the GDPR Cookie Consent plugin and is used to store whether or not user has consented to the use of cookies. It does not store any personal data. |
wsaffinity | Set by the dunnhumby website, that allows all subsequent traffic and requests from an initial client session to be passed to the same server in the pool. Session affinity is also referred to as session persistence, server affinity, server persistence, or server sticky. |
Cookie | Description |
---|---|
CONSENT | YouTube sets this cookie via embedded youtube-videos and registers anonymous statistical data. |
vuid | Vimeo installs this cookie to collect tracking information by setting a unique ID to embed videos to the website. |
_ga | The _ga cookie, installed by Google Analytics, calculates visitor, session and campaign data and also keeps track of site usage for the site's analytics report. The cookie stores information anonymously and assigns a randomly generated number to recognise unique visitors. |
_gat_gtag_UA_* | This cookie is installed by Google Analytics to store the website's unique user ID. |
_ga_* | Set by Google Analytics to persist session state. |
_gid | Installed by Google Analytics, _gid cookie stores information on how visitors use a website, while also creating an analytics report of the website's performance. Some of the data that are collected include the number of visitors, their source, and the pages they visit anonymously. |
_hjAbsoluteSessionInProgress | Hotjar sets this cookie to detect the first pageview session of a user. This is a True/False flag set by the cookie. |
_hjFirstSeen | Hotjar sets this cookie to identify a new user’s first session. It stores a true/false value, indicating whether it was the first time Hotjar saw this user. |
_hjIncludedInPageviewSample | Hotjar sets this cookie to know whether a user is included in the data sampling defined by the site's pageview limit. |
_hjIncludedInSessionSample | Hotjar sets this cookie to know whether a user is included in the data sampling defined by the site's daily session limit. |
_hjSessionUser_{site_id} | A Hotjar cookie that is set when a user first lands on a page with the Hotjar script. It is used to persist the Hotjar User ID, unique to that site on the browser. This ensures that behaviour in subsequent visits to the same site will be attributed to the same user ID. |
_hjSession_{site_id} | A Hotjar cookie that holds the current session data. This ensures that subsequent requests within the session window will be attributed to the same Hotjar session. |
_hjTLDTest | To determine the most generic cookie path that has to be used instead of the page hostname, Hotjar sets the _hjTLDTest cookie to store different URL substring alternatives until it fails. |
_lfa | This cookie is set by the provider Leadfeeder to identify the IP address of devices visiting the website, in order to retarget multiple users routing from the same IP address. |
Cookie | Description |
---|---|
aam_uuid | Set by LinkedIn, for ID sync for Adobe Audience Manager. |
AMCVS_14215E3D5995C57C0A495C55%40AdobeOrg | Set by LinkedIn, indicates the start of a session for Adobe Experience Cloud. |
AMCV_14215E3D5995C57C0A495C55%40AdobeOrg | Set by LinkedIn, Unique Identifier for Adobe Experience Cloud. |
AnalyticsSyncHistory | Set by LinkedIn, used to store information about the time a sync with the lms_analytics cookie took place for users in the Designated Countries (which LinkedIn determines as European Union (EU), European Economic Area (EEA), and Switzerland). |
bcookie | LinkedIn sets this cookie from LinkedIn share buttons and ad tags to recognise browser ID. |
bscookie | LinkedIn sets this cookie to store performed actions on the website. |
ELOQUA | This cookie is set by Eloqua Marketing Automation Tool. It contains a unique identifier to recognise returning visitors and track their visit data across multiple visits and multiple OpenText Websites. This data is logged in pseudonymised form, unless a visitor provides us with their personal data through creating a profile, such as when signing up for events or for downloading information that is not available to the public. |
gpv_pn | Set by LinkedIn, used to retain and fetch previous page visited in Adobe Analytics. |
lang | Session-based cookie, set by LinkedIn, used to set default locale/language. |
lidc | Set by LinkedIn, used for routing from Share buttons and ad tags. |
lidc | LinkedIn sets the lidc cookie to facilitate data center selection. |
li_gc | Set by LinkedIn to store consent of guests regarding the use of cookies for non-essential purposes. |
li_sugr | Set by LinkedIn, used to make a probabilistic match of a user's identity outside the Designated Countries (which LinkedIn determines as European Union (EU), European Economic Area (EEA), and Switzerland). |
lms_analytics | Set by LinkedIn to identify LinkedIn Members in the Designated Countries (which LinkedIn determines as European Union (EU), European Economic Area (EEA), and Switzerland) for analytics. |
s_cc | Set by LinkedIn, used to determine if cookies are enabled for Adobe Analytics. |
s_ips | Set by LinkedIn, tracks percent of page viewed. |
s_plt | Set by LinkedIn, this cookie tracks the time that the previous page took to load. |
s_pltp | Set by LinkedIn, this cookie provides page name value (URL) for use by Adobe Analytics. |
s_ppv | Set by LinkedIn, used by Adobe Analytics to retain and fetch what percentage of a page was viewed. |
s_sq | Set by LinkedIn, used to store information about the previous link that was clicked on by the user by Adobe Analytics. |
s_tp | Set by LinkedIn, this cookie measures a visitor’s scroll activity to see how much of a page they view before moving on to another page. |
s_tslv | Set by LinkedIn, used to retain and fetch time since last visit in Adobe Analytics. |
U | Set by LinkedIn, Browser Identifier for users outside the Designated Countries (which LinkedIn determines as European Union (EU), European Economic Area (EEA), and Switzerland). |
UserMatchHistory | This cookie is used by LinkedIn Ads to help dunnhumby measure advertising performance. More information can be found in their cookie policy. |
UserMatchHistory | LinkedIn sets this cookie for LinkedIn Ads ID syncing. |
VISITOR_INFO1_LIVE | A cookie set by YouTube to measure bandwidth that determines whether the user gets the new or old player interface. |
YSC | YSC cookie is set by YouTube and is used to track the views of embedded videos on YouTube pages. |
yt-remote-connected-devices | YouTube sets this cookie to store the video preferences of the user using embedded YouTube video. |
yt-remote-device-id | YouTube sets this cookie to store the video preferences of the user using embedded YouTube video. |
yt.innertube::nextId | This cookie, set by YouTube, registers a unique ID to store data on what videos from YouTube the user has seen. |
yt.innertube::requests | This cookie, set by YouTube, registers a unique ID to store data on what videos from YouTube the user has seen. |