Why you need a demand model
Spoiler alert
This article won’t explain how you build a demand model!
Well, it will, but only at the highest of levels. Instead, what it will do is explain why you need a demand model and what you can do with one. And knowing what a demand model enables you to do is vastly more useful and important than knowing how you build one - except for Data Science nerds like me.
What is a demand model?
A demand model is a mathematical equation that takes various relevant inputs, such as a product’s price, the promotional tactic used, prices of other similar products, and so on, and outputs the predicted sales volume. Figure 1 below summarises the inputs we typically include in a demand model.
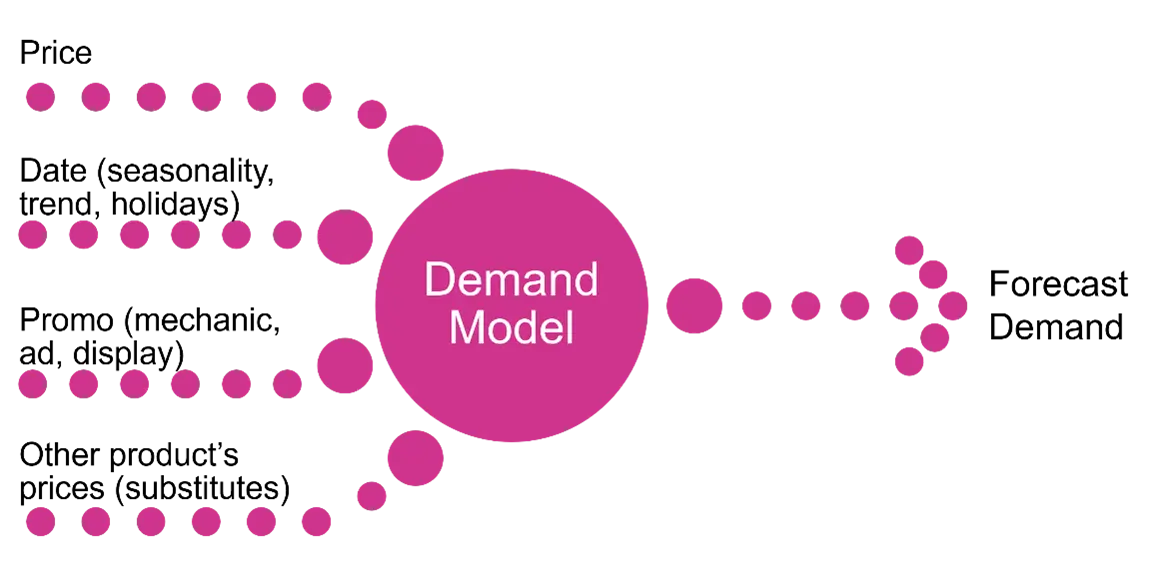
Figure 1: High-level schematic of the inputs to, and output, from a demand model
Although we use the term demand model, we are actually predicting sales volume. If supply chain issues are significant, then levels of demand and levels of sales can be different from one another.
How we build a demand model
We build our demand models just like we would any machine learning model: by taking historical sales volume data and the associated historical input values and then training the model parameters until we have achieved a good fit to the historical sales values. We typically build a separate demand model (with different parameter values) for each SKU in a retailer’s assortment.
However, we can’t just use any machine learning model for our demand model. The mathematical form of the demand model we use at dunnhumby is based on established economic and retail marketing science principles. This ensures that:
- The model output is explainable, and its parameters are interpretable.
- The model gives sensible output for any set of inputs, even for input values not seen in the training data.
Without those guarantees, we would be in danger of getting incorrect or misleading predictions in some scenarios. This would limit what we could use a demand model for. But because the output from our dunnhumby demand models is sensible and robust across a very wide range of inputs, we can safely and confidently use those demand models in lots of different ways and across lots of different situations. What are those different uses? Let’s see.
What can we use a demand model for?
Once we have an accurate and robust demand model, we can ask questions of it. Figure 1 tells us that a demand model allows us to make predictions about sales volumes. We can do this for both historical and hypothetical forward-looking scenarios. And because the demand model is explainable and its parameters directly interpretable, we can also use it to ask questions about the characteristics of the product it is modelling.
Overall, there are many ways in which we can ask these questions, such as:
- What are the price and promotional responsiveness of the product being modelled? e.g. How price-sensitive are customers to each product and so which products do I need sell at an everyday low price (EDLP)? This question is using the demand model to describe the products being sold, and so is descriptive in nature. Asking this question enables a retailer to identify optimal price and promotion strategies for each product.
- What did happen? e.g. What was the sales volume, and which driver had the biggest influence? This prediction question looks backwards in time, at historical scenarios to identify what worked well and what didn’t, and so is diagnostic in nature. Asking this question enables a retailer to identify and eliminate inefficient promotional tactics.
- What will happen? e.g. What volume will I sell over the next three months at this price? This question looks forward in time, at future scenarios, and so is predictive in nature. Asking this question enables a retailer to plan business operations ahead of time and not be surprised by what happens.
- What do we want to happen? e.g. What price should I sell each product at to make the prices attractive and relevant to shoppers and thereby increase my profit? This question aims to identify what is the best scenario that could occur and what are the steps a retailer should take to ensure it does occur. Consequently, this question is prescriptive in nature. Asking this question enables a retailer to optimise their price and promotions and so unlock extra value for themselves and their customers.
All these capabilities are underpinned by a single demand model and are summarised schematically in Figure 2.
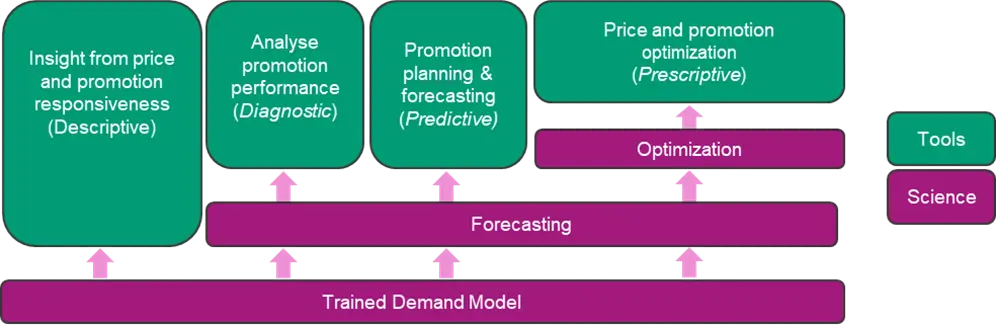
Figure 2: The uses of a demand model
At dunnhumby we have developed Price & Promotion software to perform all these tasks – from training of the demand models on historical data, through promotion planning, to price optimisation. More details on our Price & Promotion tools and solutions can be found here - our Price & Promotions offering.
Why you need a demand model
Having the capabilities shown in Figure 2 is incredibly powerful. It allows a retailer to take a data-driven approach to all their price and promotions planning.
With grocery retail margins being thin, identifying opportunities to increase margin and still be seen as price-competitive by shoppers is essential, especially in the current economic climate. Doing data-driven price and promotion planning is a must for any retailer that wants to remain competitive with cost-conscious shoppers.
And with retailer assortment sizes typically in the tens of thousands, doing data-driven price and promotion planning at scale requires expert software tools built upon robust demand models.
TOPICS
RELATED PRODUCTS
Boost value perception and execute promotions that drive results
Understand and act upon drivers that influence CustomersA look at dunnhumby’s unique Customer Data Science, which is at the core of everything we do.
Combining the latest techniques, algorithms, processes and applicationsThe latest insights from our experts around the world
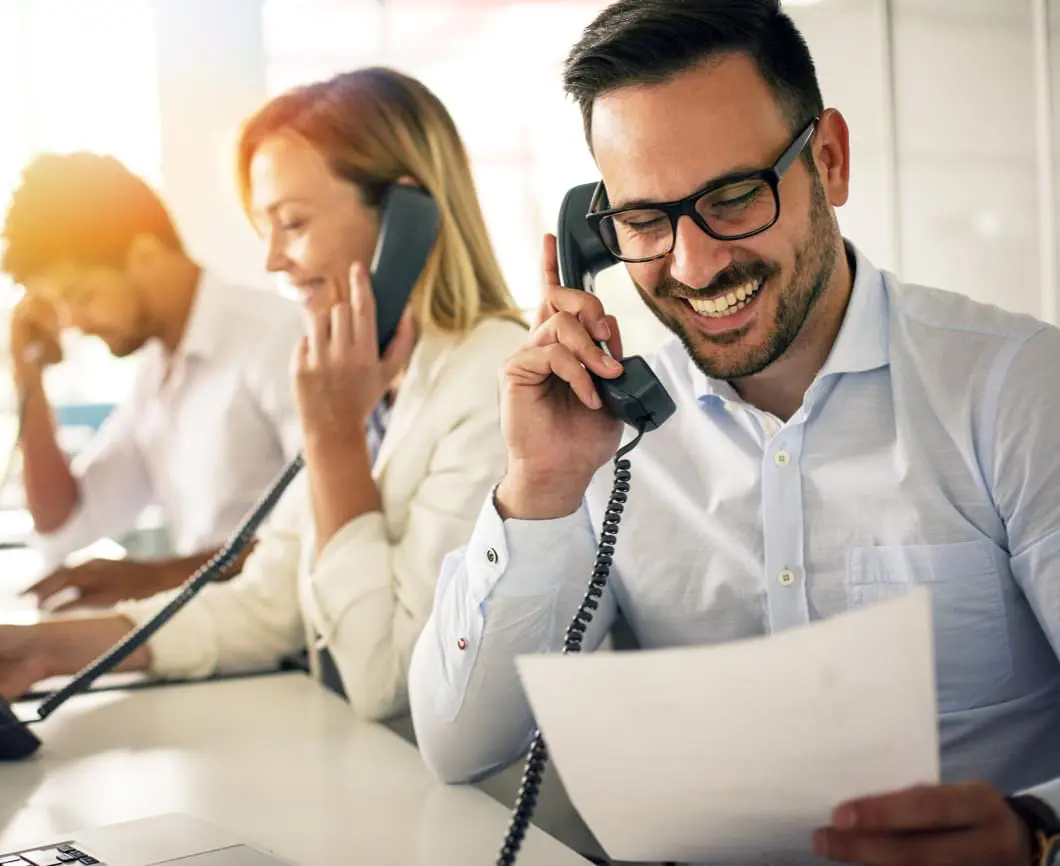