Demystifying AI
Whether or not you have spent any time online lately, you have surely become aware of a new wave of Artificial Intelligence platforms. From impressively coherent text to out of this world imagery, AI has been shaping digital experiences and is the subject of speculation from business leaders and politicians about how this technology will fundamentally change society. In the context of retail analytics, several companies have introduced new AI capabilities ranging from voice-activated interfaces for queries to bold claims about automated insights. Let’s explore what exactly AI is, how it may change our industry, and what retail leaders should expect in the coming years.
Before we delve in, it is worth noting that this is a very large, dynamic field with lots of advances occurring all the time. The goal of this article is to describe the current state of the industry as of Summer 2023 for an audience of curious beginners.
What is AI?
Artificial Intelligence is a broad category of software that aims to replicate human cognition to perform tasks. For example, facial recognition systems for unlocking phones or setting a timer with Siri are everyday instances of AI. There are several terms under this umbrella that are also important to know, including: Machine Learning, and Deep Learning, Generative Artificial Intelligence.
Machine Learning is an approach to AI that takes data from the past and training algorithms to create models that can perform highly complex tasks without being explicitly programmed.
Deep Learning is a method in AI that teaches computers to process data in a way inspired by the human brain, using neural networks to recognize complex patterns in pictures, text, sounds, and other data to produce insights and predications.
Generative AI is a subset of Deep Learning that uses neural networks to identify the patterns and structures within existing data to generate new and original content, like ChatGPT or DALL-E which produce new text and imagery. Most of the new, headline-grabbing advances have occurred in this area of the field.
It is important to note that all these kinds of AI are dependent on the training data set, the tuning of the model, and the application of the model. ChatGPT, a large language model, is well positioned to guess what words may come next in a sentence but is not designed to be accurate in the information it provides. That is, if you ask ChatGPT to create a legal brief for you with citations, it may "hallucinate" the citations to cases that do not exist.
What is new today is the emergence of these Generative AI platforms, most notably ChatGPT, which can not only find the patterns in a large data set but can also synthesize those patterns back to a user in a novel piece of content. Before this generation of AI emerged, the scope of tasks AI could accomplish was much narrower.
How is AI changing Retail?
For the data analytics firms making their first big foray into AI, we welcome you to the party. In almost every core domain, dunnhumby has some form of AI already deployed. dunnhumby Assortment uses AI to create customer decision trees and assign preliminary names to the need states, in addition to calculating the impact of adding or removing products to the set. dunnhumby Price and dunnhumby Promo both use AI in demand modelling, forecasting, and optimization of pricing and promotions. Personalization is also a key area where AI can be applied effectively. AI can be used to develop propensity models, determining how likely a customer is to try an item they have never purchased, or can be used to predict their likelihood of churning out of a retailer. Knowing information like this can fuel personalization campaigns to encourage category expansion or retain shoppers before they’ve left the store altogether.
A good use case for AI is categorizing products. Often, the process of determining where products fit in a hierarchy or determining how they are used seems simple at a first glance, but rapidly becomes more complicated as products blur category lines. For example, is Bachan’s Japanese BBQ Sauce a BBQ sauce or an Asian Sauce/Marinade? Using a program trained to categorize items can place these items rapidly, and a human just needs to review the results to ensure they make sense, as the below visual shows.
The new Generative AI models are being tested today to address a variety of challenges. With the ability to rapidly generate limitless amounts of copy writing, every customer receiving a targeted email could also get a highly personalized note thanking them for their business. Generative AI may also enable the rapid production of content like category review decks. These could be produced quickly and passed to a human analyst who can review the data to ensure accuracy and finalize the insights and recommendations.
How should retail leaders think about AI?
While the promise of rapid, actionable insights is alluring, it's important to contextualize the output from AI and ensure it’s applied to solve the right kind of problems. The above story about ChatGPT producing fictitious court cases is a great warning of the pitfalls of AI. Relying solely on an AI to produce a final, high impact decision on its own remains outside the scope of the programs today. Human interpretation, guidance, and application are still required at this stage. However, what AI can do well is to rapidly and iteratively do work that currently may take an analyst quite a bit of time - for example, quickly building several pricing scenarios or getting suggestions for different courses of action with estimated impacts or hunting for potential patterns in large data sets. AI will augment the work teams are already doing, helping them move more quickly and freeing them from busywork through automation.
There are also practical limits to leveraging AI in all parts of a business today. First, not all the knowledge in your business exists in a format that is consumable by AI, and may never be. For example, there is institutional knowledge that an AI cannot easily ingest, like contextual knowledge about a strategy shift the company undertook twelve years ago. Further, even more fundamental is the requirement of data, and massive amounts at that, to build and train these models. Without usable data on the relevant aspects of a retailer’s business, even the most advanced AI model is essentially a store with no product on the shelves. Collecting, cleaning, and storing data is a prerequisite for successful implementation of any kind of AI.
Another limitation for the application of AI is governance. Basically, to hand over key functions of the business to an AI application, users need some level of confidence in what the tool is doing. However, many more advanced models are difficult to explain. This black box approach may not be an issue in very isolated situations, like targeting customers for personalized coupons, but becomes an issue in business-critical processes.
Conclusion
Retail leaders today should be looking to AI to 1) speed up routine tasks in limited-scope domains, 2) deliver new ways of thinking about problems, and 3) identify key patterns with limited data. AI likely won’t immediately replace roles today but may be able to supplement them in a way that drives greater efficiency across the organization and provides more robust information to decision makers. There is tremendous promise, but also quite a bit of theatrics around the technology today. While it can be powerful, AI needs to be applied in context and retailers should look for partners who not only have powerful science, but also have deep industry expertise. Retail leaders need to be able to identify the difference between applications of AI that will drive their business forward from random acts of AI.
RELATED PRODUCTS
Make Retail Media work for your business with Customer Data Science
Retail Media solutionsPlan, execute, and measure the impact of omnichannel Retail Media
dunnhumby Sphere - Retail Media PlatformThe latest insights from our experts around the world
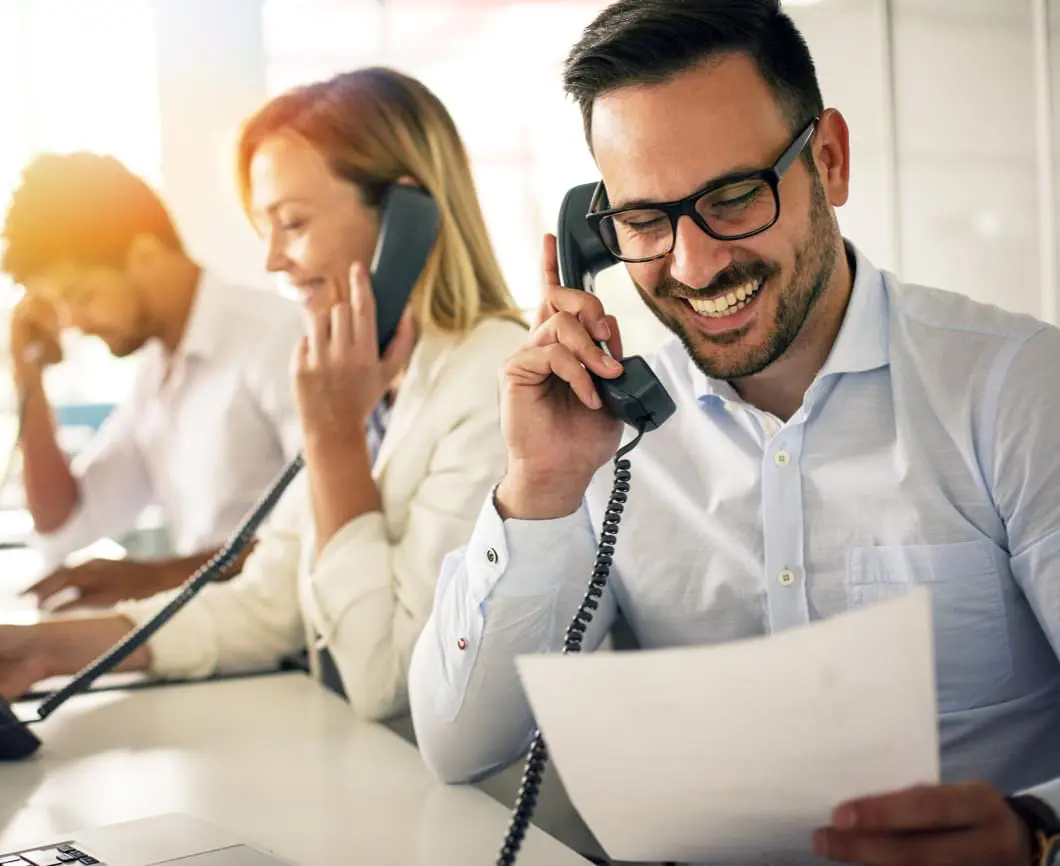