Disaggregating Forecasts
A look at different components
Act 1, Flexibility
Interior. Office. Meeting room with flashy graph on screen.
FORECASTER
“Implementing the new pricing plan will produce 30,000 extra unit sales.”
DECISION MAKER
“Interesting. How will the plan impact the sales from loyal customers?”
FORECASTER
“I’ll get back to you.”
FORECASTER
“Sales from loyal customers will increase by 20,000 units.”
DECISION MAKER
“Interesting. Will the extra sales be linked more to families with children or single professionals?”
FORECASTER
“I’ll get back to you.”
<repeat pattern until DECISION MAKER is SATISFIED or TIME RUNS OUT>
Disaggregating forecasts through a variety of lenses helps the decision maker evaluate a plan’s likely outcome before rolling it out. For a lens to be useful, its timely delivery is essential. Unless the lens is known in advance, its implementation could take more time than the decision maker has.
Because the lenses of interest may vary by decision maker, circumstance, available information and many other factors, the forecaster needs a modelling framework that is flexible enough to accommodate new lenses with minimal change to the fitting and forecasting pipelines.1
Act 2, Coherence
FORECASTER
“The total units next week will be 100.”
DECISION MAKER
“How many from loyal customers?”
FORECASTER
“70 units from loyal customers out of 150 total units for the week.”
DECISION MAKER
“How many from young professionals?”
FORECASTER
“20 units from young professionals out of 130 total units for the week.”
DECISION MAKER
“How much will we be selling in total then?”
FORECASTER
“Either 100, 150 or 130 units.”
DECISION MAKER
“Should I take the average of those figures?”
FORECASTER
“If you want to.”
The decision maker is rightfully confused by forecasts from different lenses that do not add up, when aggregated to a common level – such as overall totals. Technically, this is referred to as a lack of coherence among the forecasts.
It is well-known that models trained independently on different aggregations are unlikely to produce coherent forecasts, even if the underlying low-level training data is the same.2
Solutions to both the flexibility and coherence challenges are available.
Here, we will examine some options to consider when designing a mathematical framework for disaggregating forecasts along generic dimensions.
Reconciliation of Forecasts
In circumstances where all lenses of interest are known in advance, the forecaster could take a twofold approach:
- produce independent low-level models for each lens in advance
- funnel their forecasts through a reconciliation module.
A forecast’s reconciliation process takes a collection of forecasts and adjusts them, as little as possible,3 to enforce coherence between them.4 To do so, the adjustments to each forecast depend on which other forecasts are considered. Therefore, the reconciled forecast for a specific lens will change when new lenses are considered. Reconciliation approaches guarantee coherence only within a fixed set of lenses.
In cases where lenses are not necessarily known in advance, we need a solution that guarantees coherence between different sets of lenses too – if not, we slip back into the comedy scenario of Act 2.
Fully Bottom-Up Approach
There is always the temptation of suggesting modelling at a very low level so that all lenses of interest can be implemented simply as a sum of the lower models. Despite guaranteeing coherence and flexibility, this approach needs to be evaluated in the context of its statistical and computational implications.
If the modelling level is too low:
- each model may not have enough data to support reliable forecasts
- the computational requirements associated with estimating all the low-level models may be prohibitive.
If we want a system that is flexible enough to support coherent forecasts along arbitrary classifications of shoppers, not knowing the set of classifications in advance forces us to set the individual shopper-transaction level as our modelling level.5 This approach is untenable on both fronts:
- Statistical feasibility
In each modelling unit, there’s not enough data variation to support learning. - Computational feasibility
Even if statistical feasibility were not an issue, thinking of all the transactions taking place in a supermarket chain in two years,6 we soon realise the enormous computational challenge of having the data entering the model fitting process at such a disaggregated level.
Apportionment Techniques
A feasible approach to producing flexible and coherent disaggregation of forecasts along arbitrary lenses can rely on the interplay between two groups of models:
- Models of Units
Used to forecast the units sold at a reference level of aggregation. - Models of Proportions
Used to forecast the shares of units along further dimensions of interest.
Let’s imagine a scenario where, for a specific product, the model of units has forecasted a total 2,000 units for next week, and we are interested in knowing how many units we expect to sell to shoppers classified either as loyal, occasional or other (we will refer to this classification as the loyalty segmentation).
If we can also produce next week’s forecasted loyalty shares (for example, loyal: 30%, occasional: 50%, other: 20%) according to an independently trained model of proportions, then we are also able to produce forecasted units for each of the loyalty segments – simply by multiplying the total 2,000 units by the shares of each segment in turn (loyal: 600, occasional: 1000, other: 400). Coherence is guaranteed by construction. What about flexibility?
Choosing the appropriate models of proportions
If shares are:
- stable over time
(no trend, no seasonality, no visible dynamic) - not affected by decision variables
(for example, for a pricing decision, we would check whether shares are affected by price and promotions)
then historical averages of proportions can be a quick and inexpensive way of forecasting shares.
On the other hand, if the above conditions do not hold, averaging approaches can be misleading, and regression models of proportions with explicit dependencies on explanatory variables need to be employed.
Regression models of proportions are general-purpose models and come in many varieties: regression of empirical log-odds, Dirichlet regression, multinomial regression, and so on.7
Once the appropriate variant is identified8, it will provide flexible means to a pipeline for coherent disaggregation of forecasts.
Epilogue
DECISION MAKER and FORECASTER bond over a cup of tea.
1 Mathematical models that are appropriate at a certain level of aggregation might not be suitable for other levels. Though necessary at times, using different model families for different lenses can generate a significant resource overhead, adding to time and cost of delivering value to decision makers. In constructing a flexible analytical pipeline, model families that can cover a wide range of circumstances are desirable.
2 Any aggregation has a specific information loss associated with it, which in turn affects the noise in the estimates of the model parameters – this is in the best-case scenario where there is no model misspecification (the generating and fitting models have the same model form).
3 The reconciliation is said to be optimal if it minimises the discrepancy between the initial forecasts and their reconciled version.
4 An example of an approach to forecasts reconciliation can be found in Hyndman, R. J., Ahmed, R. A., Athanasopoulos, G., & Shang, H. L. (2011). Optimal combination forecasts for hierarchical time series. Computational Statistics and Data Analysis, 55(9), 2579–2589.
5 A single shopper is not necessarily classified in the same way throughout history - for example, at some point, they might have been a new customer, then became a regular one, and then an occasional one. Therefore, even modelling at the shopper level (pooling across their transactions) would limit the spectrum of lenses that are expressible as simple sums of the bottom level models.
6 Bare minimum time requirement for being able to estimate seasonal patterns.
7 A survey of many of these models can be found in Morais J., Thomas‐Agnan C., & Simioni M. (2016). A tour of regression models for explaining shares. Working Papers, Toulouse School of Economics (No. 16‐742).
8 Examples of elements to consider in the identification process are: is there going to be a non-negligible proportion of zeros in the observed shares? How do these zeros arise? Would a continuous model be appropriate at all possible levels of interest?
TOPICS
RELATED PRODUCTS
A look at dunnhumby’s unique Customer Data Science, which is at the core of everything we do.
Combining the latest techniques, algorithms, processes and applicationsUnlock the value of your data assets
Govern data more effectively and manage risk confidentlyThe latest insights from our experts around the world
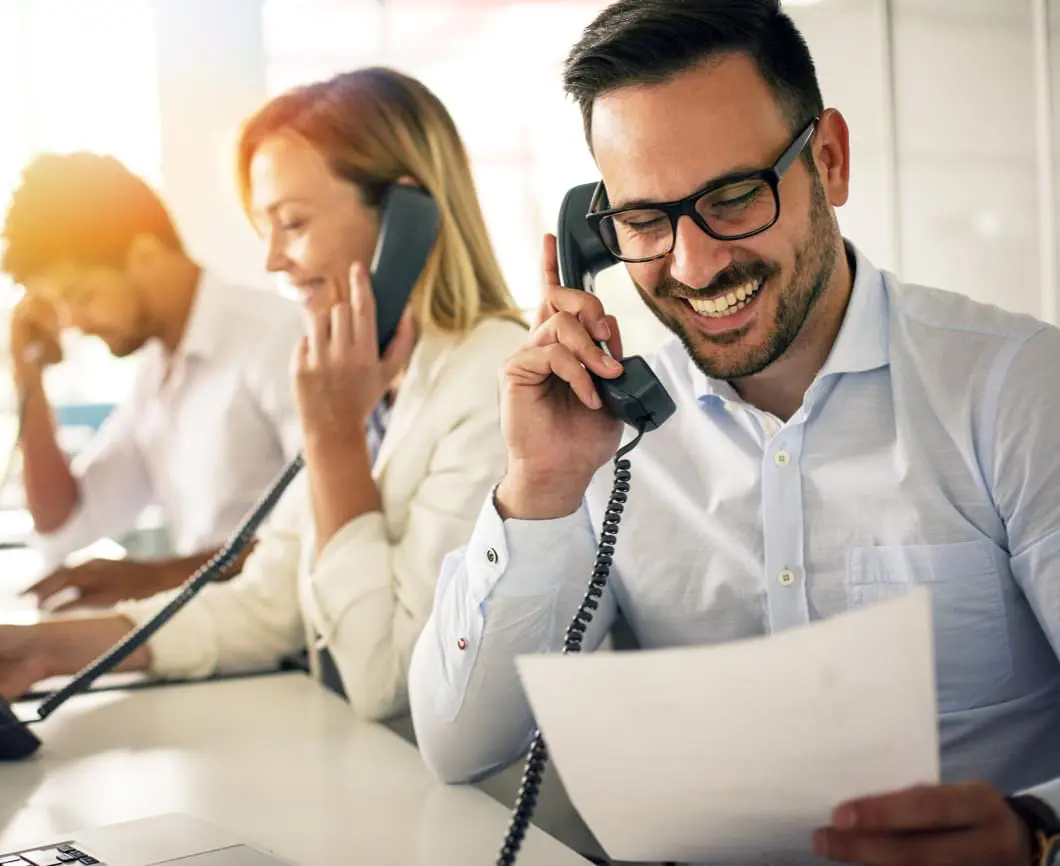