Dr. Adam Dennett: This stuff doesn’t appear on people’s radars when they’re at school
Dr Adam Dennett is Head of Department and Associate Professor in Urban Analytics in the Bartlett Centre for Advanced Spatial Analysis (CASA), University College London. CASA was established in 1995 to lead the development of a science of cities, drawing upon methods and ideas in modelling, visualisation, and computation.
I fell into a career in quantitative spatial analysis. My maths teacher was terrible, and I dropped maths at school after GCSE; It was all very pure maths, and I couldn’t really see how it could be applied. I went on to study geography and did a PhD in quantitative human geography, looking at migration and internal migration in the UK. That’s where I first came across spatial interaction models, using them to understand flows of people around the country and how these are influenced.
CASA uses spatial interaction models to understand urban and regional systems. This means flows of anything, mainly people, but also money and trade. These sorts of things govern the structure of cities, how they grow and how they organise themselves.
Data availability has changed everything. When I started doing academic research, we were using mainly census data as the source for understanding things like commuting and short-term movements. But in the last ten years data from mobile phones has started to become more widely available. Many spatial interaction models and theories have been around since the 60s, but new data sources mean we’re able to tweak and calibrate them far more effectively to get a better understanding of what’s going on.
People have been using these models in retail for years. In the early 80s two academics, Alan Wilson and Martin Clark, were working on flow models at the University of Leeds when they realised their models could accurately predict revenue for retailers.
Ford were one of the first companies to use these models to locate car showrooms around the UK. Using census data, and what they understood about the people that bought Ford cars, they built demographic profiles of people who would be more likely to spend money on a car than other profiles. They could then calibrate models to determine the best place for a car showroom, and predict, with a high degree of accuracy, that one location would be more profitable than another.
For commercial organisations who want to limit the amount of wasted money and maximise revenue, that’s quite a powerful tool. Alan Wilson and Martin Clark turned the idea into a location planning consultancy called GMAP and ended up doing retail location analysis for a whole range of big commercial organisations. GMAP ended up being one of the most successful university spin out companies.
It’s quite easy to predict what happens in large grocery stores using residential data, but it becomes a lot more difficult to predict the smaller stores. These don’t only serve local residents, but also workplace populations and people traveling through the area. Early retail modellers noticed that if you’re able to disaggregate your residential populations by types of person, then you could improve the fits of your models. It’s sprung a whole secondary industry around demographics.
The past year has made people think about bigger questions around shifting human locations, such as where people are going to be living and where they’re going to be working in future.
We’re starting to see house prices in certain parts of the country accelerating quite rapidly, yet stall in London. Big cities are the first set of indications that that maybe we’re seeing a shift. But what we don’t know at this stage is whether this is just a blip, or a longer-term trend.
In CASA, we’ve done some work looking at different working from home scenarios, from ‘everyone works from home’ to ‘no-one works from home’. We take these two extremes and simulate the sorts of impact that these have on residential demand and employment. The reality is going to be somewhere in between, so you can see what plausible scenarios might look like, if not give a definitive result.
For a long time people were not aware of the benefits of applying these sorts of models – not just the financial benefits they bring to companies – but also for urban planning and the social benefits that they can bring. If more people were able to understand the ways in which we can start to understand data through these models, then the range of applications is vast. The wider the access to this modelling, the more benefits that will be accrued by society.
This stuff simply doesn’t come on to people’s radars when they’re at school. If you look at geography curriculums, modelling doesn’t come into it. Computer science is starting to be taught in schools, but it’s quite functional. Yet when you look at the job market, data scientists are needed everywhere, particularly in local and central government, using data analysis methods to enhance societies.
Education is the real opportunity to democratise and provide wider access to spatial analysis. At CASA, we don’t care about your background; if you’re interested, we’ll teach you enough to pull some code from GitHub and start to explore and adapt it for your own uses.
We need to get into schools and to show people that someone like me, who hated maths at school, has made a career out of data modelling. That’s our next challenge in CASA, and that could be quite powerful.
TOPICS
The latest insights from our experts around the world
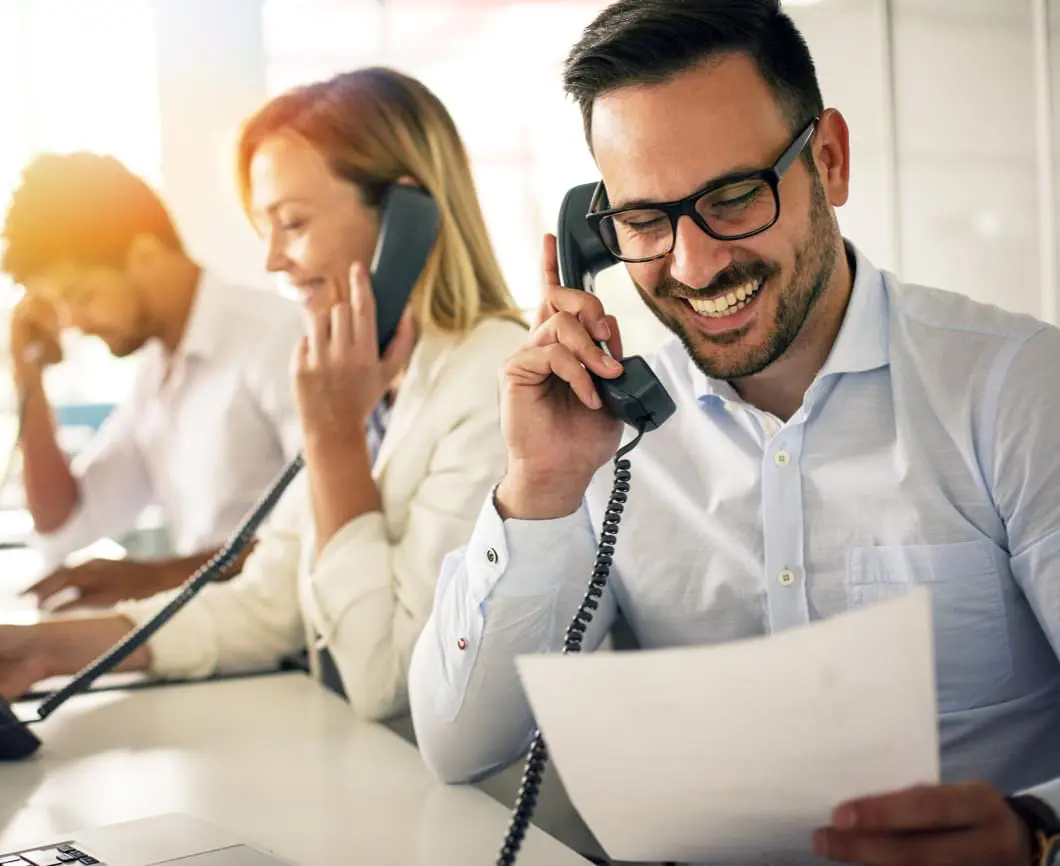