Gravity modelling and grocery sales: how customers decide where to shop
With so many different brands, formats and channels to engage with, deciding where, when and how to do your grocery shop can be a complex decision. Some of us will do a regular weekly shop at a large superstore, some will do smaller top-up shops throughout the week, and others will use their mobile to order groceries to their home at a designated time.
As strange a question as it may seem, can the idea of gravity be used to account for all these different behaviours?
In this article I summarise some of the findings from my recent Advanced Spatial Analysis PhD Thesis conducted at the Centre for Advanced Spatial Analysis UCL, and in partnership with dunnhumby. I’ll look at the idea of gravity modelling in retailing, and how the model can be used to estimate grocery store sales in the UK.
- What is gravity modelling?
Gravity is a concept most of us will have been taught at school. You’ll likely recall the story of Isaac Newton sitting under a tree when an apple fell onto his head, leading him to wonder why the apple fell straight down as opposed to sideways or even upwards. This line of questioning eventually led to Newton identifying gravity as a force that holds us on the ground and keeps the moon and planets in their orbits.
This idea was then linked to the movement of individuals by Ernst Georg Ravenstein in the late 1800s, and William J. Reilly was the first to link gravity to retail. Reilly formulated an equation that could be used to determine trade boundaries between different cities based on the idea of gravity. This would be the point at which an individual would be indifferent to visiting city A or city B for shopping, helping retailers and city planners to determine where best to locate retail outlets.
- Modern applications
While gravity modelling has been adapted and improved upon since Reilly’s time, the central concept remains fundamentally unchanged: the flow of goods between two locations is proportional to a measure of the size of the origin and destination, and inversely proportional to the distance between them.
In grocery, the key factors at play are the amount of money available to spend from each point of origin, the attractiveness of the store, and the travel time between them.
Most modern implementations of the gravity model in retailing now take the form of the model derived by A. G. Wilson, known as the “origin-constrained” or “retailing” model. This takes advantage of the mathematics of entropy-maximisation from thermodynamics, placing the model on a more robust mathematical footing and allowing for easier calibration.
To calibrate this model, we can use anonymised loyalty card data which shows the total amount spent per origin at each destination within a given timeframe. The calibrated model can then be used to predict total store revenue based on the estimated revenue available from each origin.
- Modelling a region
Previous applications of gravity models using loyalty card data suggested that they could be highly accurate. At a small spatial scale they could be used to predict store revenue to within +/-10% across a whole year. Our research thus began with attempting to scale these models to predict store revenue for a whole region within the UK, essentially estimating the revenue for over 100 supermarket size stores for each week across a whole year.
The gravity model was thus trained on a dataset of anonymised loyalty card data from a national grocery retailer. This data showed the amount spent per week, per output area (a UK census area with around 150 households), and per store. The calibrated parameters from this model were then used to estimate total store revenue for the whole region, which could then be compared to the actual revenue.
What our results showed, however, was that at this scale the gravity model was unable to account for the variation in store conditions and consumer behaviour to reliably predict actual revenues. While a few stores were well predicted on a consistent basis, the majority were poor enough predictions that the model would unlikely to be used in practice to make new store location decisions.
- Replicating previous results
The next step was to see whether we could replicate the performance seen in previous papers. These papers suggested that a gravity model could be reliably used to estimate grocery store revenue to within +/-10% across a whole year for four stores, and +/-30% for sixteen stores. Perhaps we had just been modelling too many stores all at once.
To make sure that our attempts at replication were consistent, instead of using a single group of either four or sixteen stores, we replicated the model across 47 different groups of each size. These stores were selected from a single region based on stores that were located next to each other, replicating the conditions from the previous papers.
Our results from this analysis showed that while there could have been conditions that led to the performance seen in the previous literature, their results could not be consistently replicated. This was such that while a single group of each size showed results within the previous bounds, the other 46 groups did not.
These results supported the argument from the regional model application that – at this scale, and with the current data and modelling formulation – the gravity model was unable to account for the variance in underlying store characteristics and consumer behaviour at regional scale.
- Alternative models
This left two questions: was it the form of the model that was affecting performance, or was it changes in behaviour by consumers that meant that a gravity model was too simple?
To answer the first of those questions, we employed some alternative models. Adaptations here included:
- A model exploring competing destinations and two-level decision making.
- A model that included the age of a store as a measure of attractiveness.
- A model that aimed to reduce the influence of convenience and multi-purpose trip behaviours on results.
None of these models showed any significant performance improvements, however, leading us to our final line of questioning.
- Modern shopping trends
Our results suggested that either an important feature was missing from the model, or that behaviour had fundamentally changed from what it was before. This took us to the issue of the shopping trends that have emerged since the beginning of the 21st Century in the UK such as:
- Convenience Shopping – characterised by more people shopping within a much smaller travel time, shopping with a greater frequency, and doing so at “convenience stores” of big retailers.
- Rise of the deep discounters – continental discount stores began to muscle their way into the UK market from the 1990s but saw some of the largest gains in market share in the 00s and 10s in response to a perceived gap at the bottom of the market.
- Online shopping – the adoption of e-commerce in grocery retailing has had a significant effect on the geography of grocery retailing. Distance is no longer likely to be seen the same way and the size of the store is unlikely to be the main driver of attractiveness.
These changes in both consumer and retailer behaviour can be seen to influence interactions within the grocery retailing market. This affects the underlying assumption of the gravity model that shopping would be undertaken on a weekly basis, with consumers travelling by car and with a clearly defined regular basket.
This may mean that for the gravity model to reach levels of accuracy seen before, it would need to adapt or potentially that newer models such as Random Forests, Neural Networks and Agent Based Models (ABMs) need to be used.
This is a highly abridged version of an article that was originally published on Medium.
TOPICS
RELATED PRODUCTS
Make Retail Media work for your business with Customer Data Science
Retail Media solutionsPlan, execute, and measure the impact of omnichannel Retail Media
dunnhumby Sphere - Retail Media PlatformThe latest insights from our experts around the world
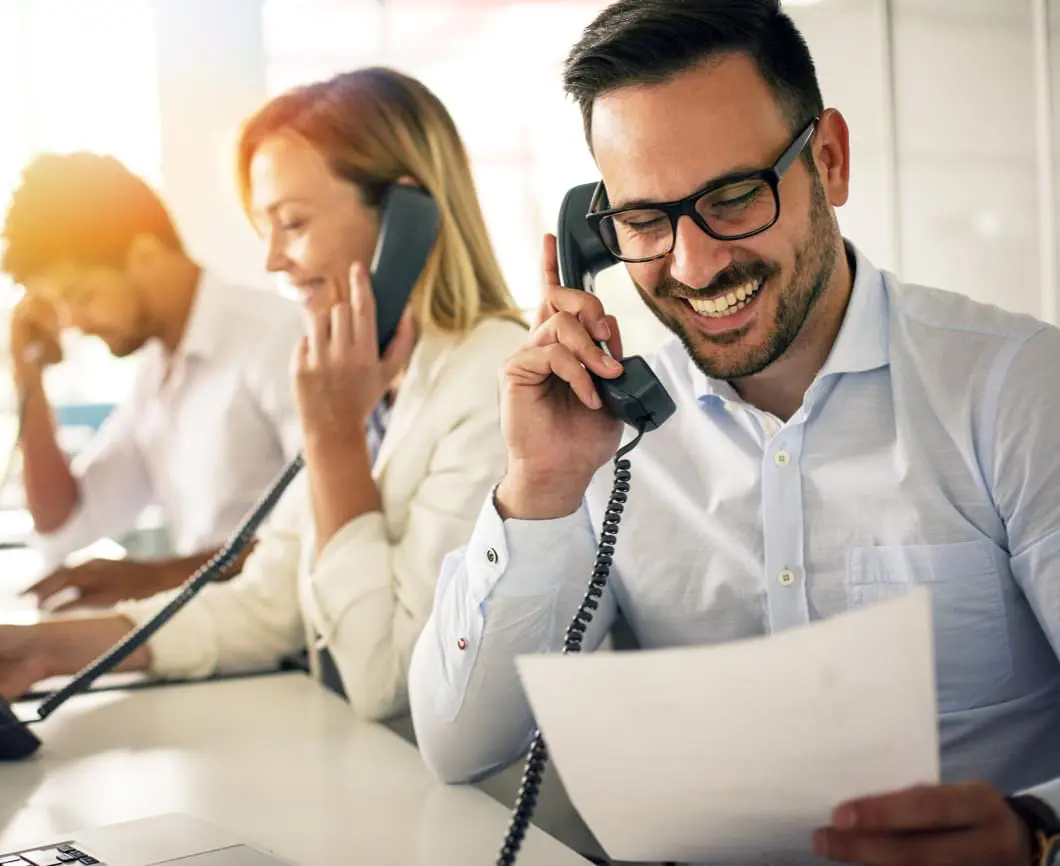