Topic modelling: how a statistical technique can help us better understand customer missions
Let’s talk about baskets.
When we look into a customer’s basket, we can tell a lot about them. Those observations can range from the simple, like what they’ve bought, where, and when, to the more complex such as why they came to the store in the first place and connections between the different items they bought. With the right approach, we can even predict what they might buy next. Either way, the better we understand their baskets, the better we understand customers too.
At dunnhumby, that understanding starts with “Trip Missions”. These are basket-level segmentations that tie multiple need states together in order to understand a customer’s main reason for visiting a store. Trip Missions are key, because they can help retailers understand business performance and the effectiveness of their pricing, assortment, and store experience, as well as giving them the ability to tailor messaging to specific missions.
In order to create these segmentations, we also have to find out what those different need states are. The only way to do that, of course, is to dive deep into customer baskets, look at what they’re buying, and conduct the analysis required to single out need states and combine them into meaningful missions.
The good news is that there’s no shortage of baskets to peer into. Retailers around the world process billions of baskets every year, and dunnhumby Shop – one of our key insight and analytics tools – analyses well over 20bn baskets per annum on its own. The bad news is that turning that data into something that helps us understand common behaviours across millions of customers is no simple task.
While dunnhumby already has numerous ways of approaching that challenge, we’re always on the lookout for smarter and more sophisticated techniques as well. That’s where topic modelling comes in.
What works for documents can work for grocery too
Now, topic modelling isn’t something that’s specific to either the grocery industry or dunnhumby. It’s a statistical technique that’s most commonly used for finding “hidden” topics that can be used to describe a collection of documents. Topic modelling is frequently used in conjunction with automated text-mining, allowing large collections of documents to be understood in a systematic and approachable way.
Topic modelling is also a form of “unsupervised learning”, and approach that applies machine learning processes to the analysis of untagged or unlabelled datasets. The primary benefit of unsupervised learning is that it helps us find commonalities and significant differences within a dataset, all without a human needing to guide things.
Because of that, and while it might not have originated within the grocery industry, topic modelling has clear relevance – particularly when we apply it to the analytical conundrum outlined above. Just as it can be used to find common themes across millions of documents, topic modelling can also be used to identify “basket topics”, those need states satisfied by a certain set of products.
In many ways, the exact same principles that apply topic modelling within text-mining can also be used during basket analysis. Rather than reinventing the wheel, we simply need to substitute those aspects that are specific to text-mining in this process with those that relate to grocery.
Take a look at the diagram above, for instance. Here, we’re using the same modelling techniques that we would during text-mining to sort our four different “documents” – or, baskets – into different “topics”. Rather than just being a group of seemingly disconnected products, each basket is now aligned to a bigger theme. Naturally, that’s incredibly powerful when you extrapolate that across hundreds of thousands, or even millions of baskets.
Identifying common missions
The next question, of course, is where these topics themselves come from. Naturally, while there’s an academic answer to that question, the best way to think about it from a grocery perspective is in terms of customer needs. Essentially, topics are created around groups of products that are frequently bought together and fulfil a specific customer need. A “dog products” topic might contain kibble, wet food, chew toys, and training treats, for instance.
Let’s just wind back to our original goal here. As mentioned towards the beginning of this article, what we really want to understand is Trip Missions – basket-level segmentations that help us understand why customers are coming to store. Topics are incredibly useful in this context, because they can be used to identify common Trip Missions across different baskets through the process of clustering.
Again, a diagram is useful here. In the example below, we have a collection of overarching Trip Missions and supporting sub- (or low-level) missions. Each of those sub-missions has been identified by looking at topic clusters. These then feed up into that high-level mission; in the first column, for instance, “Scratch Cooking”, “Breakfast”, “Cooking on a Budget”, and “Quick Meals & Snacks” combine to form the “Food at Home” Trip Mission.
Understanding topics – and how they ultimately shape customer Trip Missions – is an immensely useful capability for a retailer to have. Whether it’s trend analysis as part of KPI reporting, a better understanding of assortment and category flow, or being able to bundle key items around seasonal events, Trip Missions can be used in a multitude of ways to refine and improve performance.
Ultimately, topic modelling provides an efficient and effective way of understanding data at the kind of scale required within grocery retail. As mentioned above, drawing consistent insights from billions of baskets is no easy task. Unsupervised learning techniques like topic modelling help us understand what customer baskets are telling us, without us needing to add our own assumptions about their behaviours into the mix.
For retailers, topic modelling gives them the ability to understand shopper missions with greater certainty, ensuring that they can respond with the right tactics across everything from pricing and assortment through to media and customer service.
TOPICS
RELATED PRODUCTS
A look at dunnhumby’s unique Customer Data Science, which is at the core of everything we do.
Data Science solutionsThe latest insights from our experts around the world
The six drivers of private brand innovation
AI's Regulatory Crossroads: Innovation vs. Control
Why you need a demand model
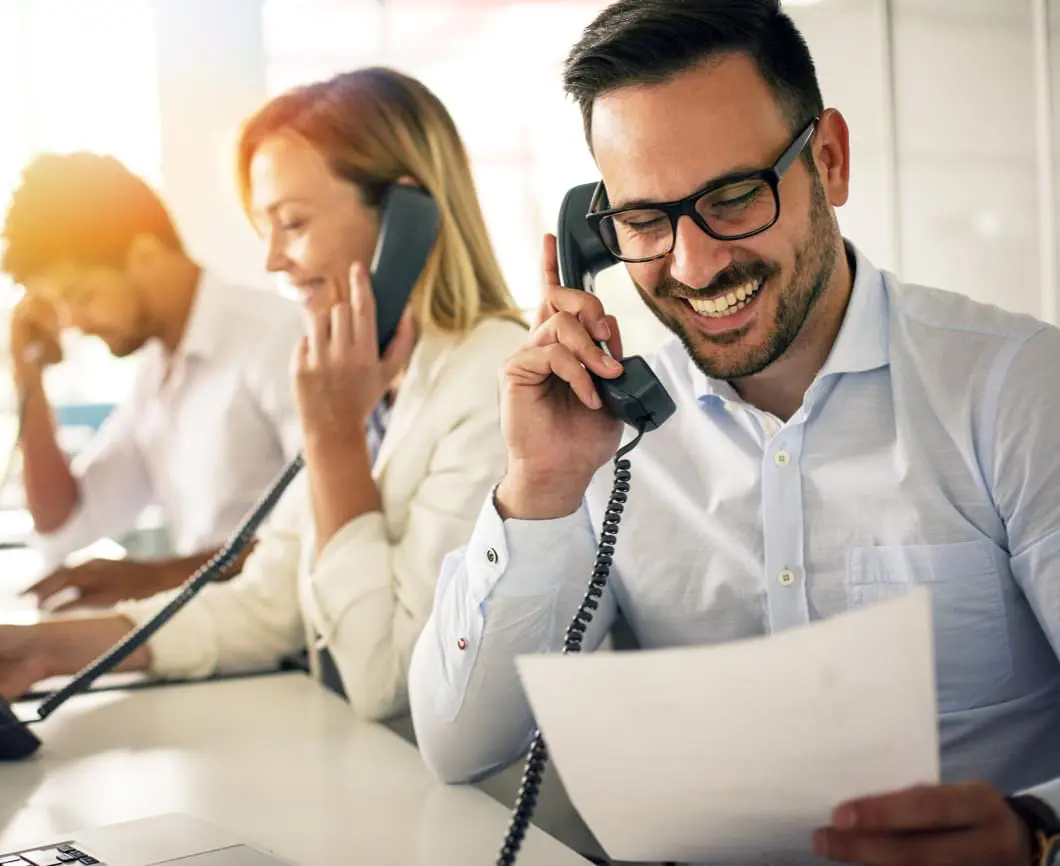
Speak to a member of our team for more information