The application of customer data science (analysing customers’ purchase behaviour) is the backbone of modern retailing. Knowing more about your customers’ needs, wants and shopping habits helps deliver a better shopping experience, leading to an increase in loyalty, and growth in sales, through mechanics such as the right range in stores, meaningful and relevant communications, optimised pricing on product lines and promotional offers.
There is much debate about what is the ‘right type of science’ to identify the right customers. Which are the best methods for determining who will buy your product, who will respond to your promotional offer? Marketing budgets are being squeezed for retailers and for brands, so it’s more important than ever to get it right with your targeting, and at dunnhumby we’re constantly looking for new ways to help retailers and brands delight shoppers and improve their performance by putting the customer at the heart of decision making.
Current targeting methods rely heavily on a rich purchase history. Take a typical promotional coupon campaign: one way to select your audience would be to identify customers who have frequently made purchases relating to the category or product on offer. While patterns of past behaviour can be a good indicator of future likelihood to purchase, they do not account for potential new shoppers who might never have purchased that brand or category before but might be motivated to trial it.
As part of my PhD, I set out to investigate if network science could be successfully used as an alternative approach to targeting that might yield better results. Network science focuses on the interaction and relationship between things. Twitter is a good example of network analysis in practice, seeing for example who are the key users in spreading a viral news story.
Applying network modelling in a retail environment allows analysis of connections between customers and products where there was a purchase. Densely connected clusters or ‘communities’ that are detected algorithmically in these networks reveal customers with similar preferences and the products that they buy the most. ‘Missing links’ within these communities then form the basis of new product recommendations. This approach differs from supervised machine learning in that there is no requirement to define any features about customers, for example you don’t have to look at what they spend in various categories. It picks up the patterns in the data in a more hands-off way, without having to define the inputs into the model.
An example of a network showing how customers and products relate to each other in ‘communities’.
Networks use transaction data directly to infer loose connections between customers and products, and these connections are the key to a new approach to targeting.
To test how this network modelling method compares to traditional targeting, we applied the approach to a promotional campaign in the yoghurt category. The control was an existing coupon campaign which used a targeting approach based on a combination of previous engagement with the product on promotion and possible engagement with competitor products. Customers were then prioritised according to how much they spend in the yoghurts category overall. We wanted to see if using a networks’ approach can better pinpoint those customers who were most likely to redeem the coupon.
The original campaign involved a promotional offer mailed to 100,000 customers. We used this data to compare two ways to rank customers in terms of how likely they are to participate in the promotion. If higher-ranked customers redeem coupons more often than lower-ranked customers, then the ranking has predictive power.
The results showed that the ranking based on the network model was more useful than category spend at picking out relevant customers to target, as it yielded a higher redemption rate. The gap between the two rankings was especially pronounced for ‘lapsed’ customers, whose purchasing of the promotional product declined in the time period leading up to the campaign. More testing is required, but the initial results are encouraging.
While there are pros and cons to using network science (one of the downsides is the amount of computing time/power required to find meaningful communities in networks of millions of customers and tens of thousands of products), developments in technology platforms are enabling data scientists to work with bigger and more varied data sets, so this may be less of an issue in the future.
In the race to understand customers’ needs, wants and purchase behaviours to create a better shopping experience, the scope for use of network science in retail presents the industry with some exciting possibilities.
Roxana Pamfil is a former PhD student at the University of Oxford, working in collaboration with dunnhumby as part of the EPSRC Centre for Doctoral Training in Industrially Focused Mathematical Modelling. In her research, she developed network algorithms for analysing consumer behaviour. She is currently a Knowledge Transfer Ambassador at dunnhumby, funded jointly by the University of Oxford and dunnhumby, implementing research findings within the business.
The latest insights from our experts around the world
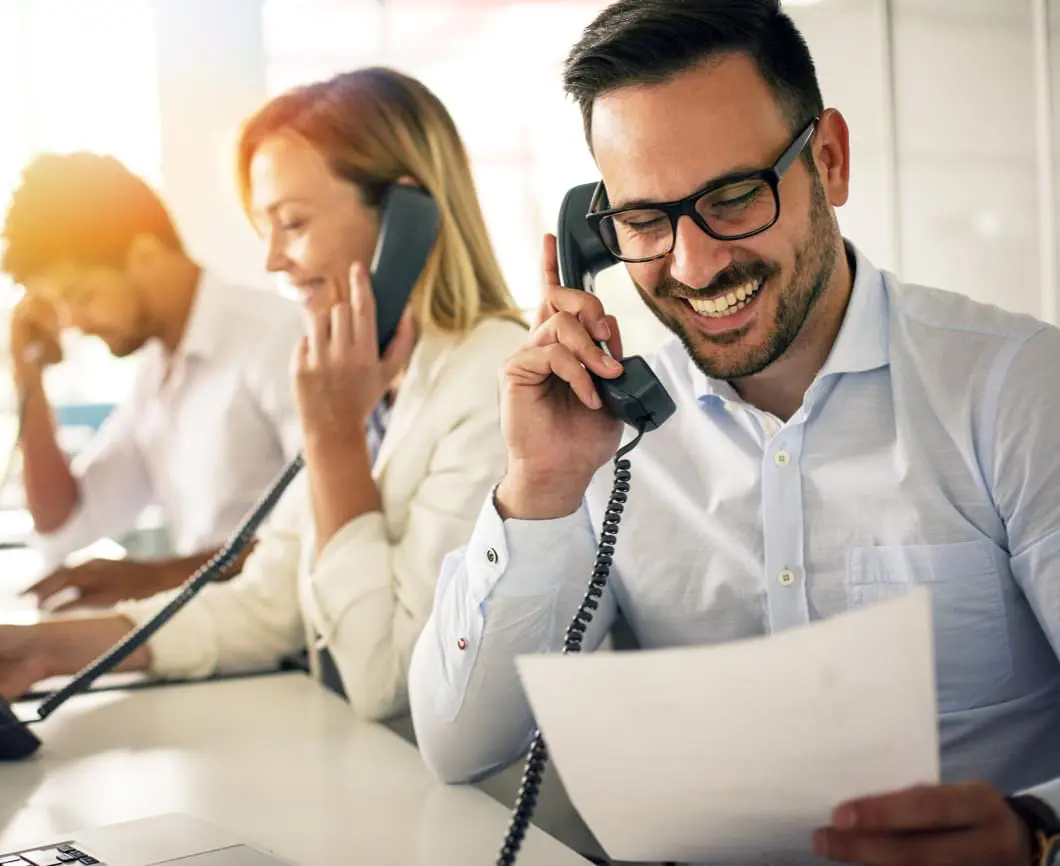
Speak to a member of our team for more information