A strategic approach to data enrichment
How much data is too much? Obviously, the answer to that question depends on the context in which it’s being asked. When we look at enterprise data specifically though, one thing becomes clear quite quickly – more isn’t always better.
Over the past few years, a growing number of organisations have begun falling into the trap of believing that the more data they collect, the better insight they’ll end up with. Consequently, they end up hoarding all kinds of data in the hope that it will – somehow – help them steal a march on the competition. Compounding that is the presence of certain technology vendors, who are only too happy to support those ambitions.
The exact shape that this “support” takes can vary wildly, from data-on-demand and data workbenches through to collaborative clouds. Once the shopping spree is over, however, buyers tend to find themselves struggling to integrate those technologies effectively or realise a return that comes anywhere close to the vision that they were originally sold on.
One of the fundamental problems here is the (usually) cloud-based nature of that technology. If data storage capacity were unlimited and computation cheap, data hoarding could deliver at least some value. With most services demanding cloud services on a pay-per-use basis, though, costs mount in line with the volume of data that is being stored, accessed, and analysed.
That’s just part of the problem, too. The more sources and nodes that organisations add to their base layer of data, the more that management and quality control issues tend to arise. As a result, accuracy is undermined, representativeness suffers, computation time increases, and the ability to take advantage of new and emerging opportunities typically falls away.
To prevent this from happening, organisations need a strategic approach to data enrichment – one that systematically brings in only that information which is most relevant to the task at hand.
Deliberate vs. emergent data strategies
In their 1985 paper “Of strategies, deliberate and emergent”, business scholars Henry Mintzberg and James Waters wrote about the nature of strategic choice. In it they identified two strategic philosophies, the eponymous “deliberate” and “emergent” approaches.
In Mintzberg and Waters’ eyes, a deliberate strategy can be defined as one that arises from the conscious, thoughtful, and organised decisions taken by a business after the rigorous analysis of data. An emergent strategy comes from spontaneous actions and initiatives, often as an outcome of innovation or in response to unexpected opportunities. When compared to a deliberate strategy, an emergent strategy allows for a higher degree of flexibility, allowing organisations to adjust goals and pursue opportunities as they emerge.
While it may sound like these are opposing approaches, that’s not really the case. If we think about an organisation’s strategy as a linear process, then the deliberate aspect of that is simply the original intent: what was “meant” to happen. The emergent part then takes into account any new factors and issues that have come to light along the way, and how the organisation responded. They’re two parts of the same journey.
Why does this matter? Because, rather than choosing between deliberate or emergent when it comes to the enrichment of data, I think it’s more important to find the right balance between the two. To be truly successful with their accumulation and management practices, organisations need to be able to blend deliberate and emergent strategies and understand how to employ each when it is most relevant.
As with my opening question, context plays a key role here; what works for one organisation won’t necessarily do so for another. That said, I do believe that there are at least some universal guidelines that can be applied in order to ensure the greatest likelihood of success. With that in mind, here is what I believe is a five-step model for the creation of a well-balanced data enrichment strategy.
1. Create a strong foundation
A deliberate data strategy is the rock on which everything else should be built. No matter how agile they may wish to be long term, organisations still need to establish a Single-Source-of-Truth (SSOT) hub that builds up a reserve of ethically and legally sourced data over time.
2. Build with purpose
While I might have characterised data hoarding as a problem above, that’s not the case when it comes to data that is purposefully and selectively curated. Add “related” nodes of relevant data with specific use cases, ensuring overall fit with the SSOT hub for scaling later.
3. Eliminate any bias
Interrupt and weed out any input data bias during the enrichment and integration phases. Bringing in rich demographic data that covers a fraction of the total customer population could add more features to the data model but also introduce bias, for example.
4. Start with small-scale experiments
Conduct preliminary data investigations and experimentations on thin slices of representative sample data. After testing your models and removing any biases, scale the underlying data to make your solutions ready for production.
5. Optimise, optimise, optimise
Not all data will be useful – and even information that is useful initially can age out of your models. Establish strong bottom-up controls that detect and purge redundant data, ensuring that you can optimise your cloud storage and compute costs while improving the accuracy of your analyses.
Executed well, this approach will deliver myriad benefits – not least:
- Better cost control across the data and value-delivery chain.
- High quality, relevant, and connected data that powers accurate insights and meaningful recommendations.
- An ethical approach that provides better control over bias.
- Improved AI models and explanations.
More than anything, though, a balanced approach to data enrichment gives organisations the ability to build a sustainable first-party data asset that can be scaled and managed economically. Therein lies the path to that all-important return on investment – and genuine competitive advantage as well.
TOPICS
RELATED PRODUCTS
A look at dunnhumby’s unique Customer Data Science, which is at the core of everything we do.
Data Science solutionsThe latest insights from our experts around the world
Disaggregating Forecasts
The six drivers of private brand innovation
AI's Regulatory Crossroads: Innovation vs. Control
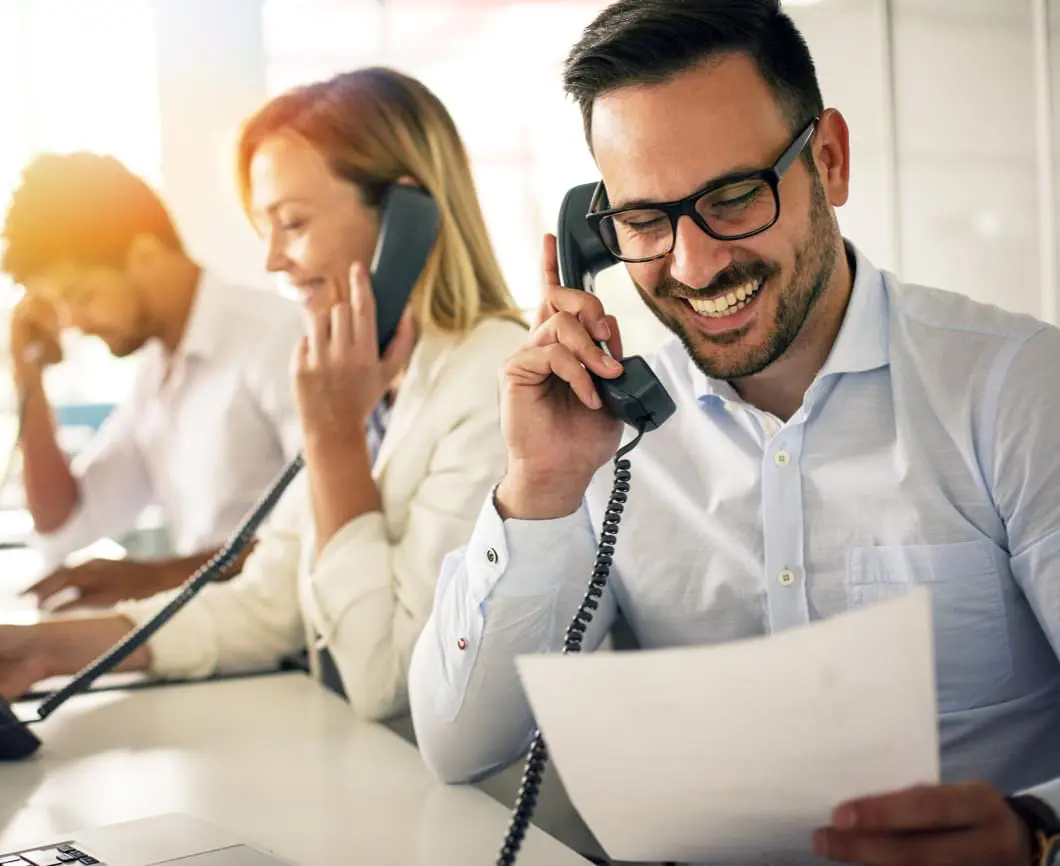
Speak to a member of our team for more information