Data Ethics: the emperor’s new clothes?
Last year I took on a new role at dunnhumby, leading our best practice for data management and governance. As part of this remit I knew I needed to assess our position on the hot-new-topic of Data Ethics, deciding where we were doing well and where we need to focus and improve.
The first step was to understand what was meant by the term ‘Data Ethics’. This terms, alongside ‘AI Ethics’ has become a hot trend, with articles, roles, software and whole companies springing up to help data-rich organisations tackle this. The use of the word ‘ethics’ creates a weight to the topic, a sense of obligation and risk. No one wants to risk being branded ‘unethical’, but it’s not immediately obvious how one avoids this; this goes beyond being legal/privacy compliant.
For me, Data Ethics is about what we consider when we decide a) what data to use, and b) how to use it, to ensure we are treating our clients, their customers, and our colleagues with respect. As a customer data science company who transform and analyse billions of data points every day, Data Ethics is not something we take lightly.
Some organisations are ahead of the game and I am very happy to learn from them. For the past few months I have had the Open Data Institute’s ‘Data Ethics Canvas’ printed out and stuck on my wall. It is a stunning visual, and evokes the image of an information and process tsunami and has left me questioning where to start and how much there is to do? It’s easy to feel like a very small person at the bottom of a very large hill.
But as I have started to dig into this topic, I have been relieved to discover this is not a whole new data discipline. In fact, many questions are the same ones that data engineers, CTO, CIOs and data governance teams have been facing for years, and more recently infosec and privacy lawyers. You could consider these aspects the ‘hygiene factors’:
- Do I know what data I want to use and what the level of quality is?
- Do I have the right permissions to use it?
- Can I secure the data from accidental or malicious leak/theft?
For many of us in the data science industry, this stuff, although sometimes complex to answer and implement, is our bread and butter; they are the questions we ask every day to ensure we are compliant with legislation and organizational policy.
And so it’s reasonable to ask, is the buzz around ‘data ethics’ justified? Or is it the emperor’s new clothes? Have we all been doing this already?
More challenging questions
But then we come to the really challenging stuff; the questions that make your brain hurt and have you exploring worm holes at 3am, and many of these questions are indeed fairly new to our industry. Many are a natural extension of the hygiene factors above, but they pose a much more challenging, complex and open-ended set of questions:
- What are all the potential consequences on society of the data I am using and how I apply the insights I have derived from the data?
- Is my data limited in a way that impacts my understanding and application of insights derived from it?
When it comes to industries that regularly generate and use highly sensitive, highly regulated data, such as health care and banking, examples of the above spring to mind easily. But even in the seemingly innocuous world of retail we should now be asking these much broader questions.
Let’s look at some examples…
- What are all the potential consequences on society of the data I am using and how I apply the insights I have derived from the data?
At dunnhumby, when we consider a new way of using data, we usually focus firstly on how this can benefit the shopper – how can their experience of the retailer be improved through the use of data and data science. This might be about offering a better curated range that suits their needs and preferences, it could be about making the check-out more convenient, it might be about how to inspire them based on products they have browsed or previously bought. This will be explicitly linked to the retailer’s strategy e.g. are they trying to grow a certain category or launch a new own-brand range.
Most companies would stop there, confident the objectives will be met, and proceed with the work. What often doesn’t happen is a more holistic assessment that considers the unintended consequences from the use of data – the knock-on effects, the groups that are indirectly impacted, the potential misuse of data or insights. At dunnhumby we have a data governance board designed to debate and decide upon these scenarios, and we are increasingly getting into these holistic assessments.
In recent months, for example we have discussed the potential implication of joining up loyalty card transactions for multiple members of the same household (something considered common practice in many countries). The benefits being that we creating a more realistic view of the consumers of products, and have the ability to understand how tastes and preferences impact the entire household shop. We may also use this to streamline communications to that household, so they get one relevant message, rather than duplicates to each individual member. All sounds sensible and beneficial to the shopper. But there are potential downsides; could the ‘relevant communications’ expose purchases by one household member to another? Could we fall into assumptions about the make-up of the household based on societal expectations?
Another example that is often debated is the use of demographic or profiling identities. This has been common practice across the world, with features such as age, gender, socio-economic band still regularly used to segment and target customers, and in many countries going beyond this to look at ethnicity and religious affiliation. But how much does the use of these labels help reinforce harmful, out-of-date stereotypes, and limit our ability to challenge and change these? For the past 30 years, dunnhumby have focused on analysing what people are really doing, what they are really buying and using that to improve and tailor their experience with retailers. We strongly believe what you have purchased previously is a much better indication of what you might purchase in future than demographic information and helps us avoid potentially harmful stereotypes.
- Does my data limited in a way that impacts my understanding and application of insights derived from it?
Traditionally when we consider data quality, we want a complete, timely, accurate data set. In the world of retail this often means a data set that contains all the till transactions for a recent period, and ideally a link to a customer database created through marketing activities such as a loyalty programme. In this scenario ‘complete’ data could still be missing vital behaviours or groups of customers who represent sections of society because they are not accessible through the retailer’s data.
An example would be a traditional loyalty segmentation that considered how much a customer has spent in assessing their ‘loyalty’ (RFV; recency, frequency and value). If loyalty is truly what we want to understand, the important data is their share of wallet, or the number of categories they are shopping. If we go on total spend alone we can easily be excluding customers who are spending less overall which could be driven by many factors, not necessarily their loyalty. The unintended consequence of this could be that those customers are missing out on the best offers and coupons, despite being loyal to the retailer. The reason that they are spending less could be a consequence of being in a lower income bracket, and so we are starting to uncover some potential unintentional bias or even discrimination.
Another example would be the very use of a loyalty card to capture transactional data to analyse. Many retailers will be making significant decisions about how to best serve their customers through this dataset, however there could be whole communities that are not represented because they chose not to use loyalty cards for a variety of reasons.
Conclusion
It’s become clear to me that Data Ethics is not the emperor’s new clothes; it is an evolution of data security and data privacy and it is bringing new considerations and challenges to the data science industry. This is partly driven by government and legislation, but also by citizens’ expectations.
In the world of retail loyalty, we often talk about a ‘value exchange’ – this used to be limited to tangible monetary reward for sharing data (e.g. if I use my loyalty card, I get some relevant coupons), but it is going beyond this; people now expect their data to be used ethically and even to contribute to improvements across society.
It is an incredibly challenging area for data rich companies; there is no binary answer to whether an action, a use of data, a data set is ‘ethical’. The most we can do is establish solid frameworks to assess the scale of impact on individuals and set parameters that allow our data science and data engineering communities to work and innovate within while minimising risk.
The key for me is that we continue to challenge ourselves and debate these topics. There will be many times that we cannot avoid some unintended consequences or bias, but what is crucial is that we do this knowingly and thoughtfully and use these experiences to continuously improve how we use data to provide benefits.
TOPICS
RELATED PRODUCTS
A look at dunnhumby’s unique Customer Data Science, which is at the core of everything we do.
Read moreThe latest insights from our experts around the world
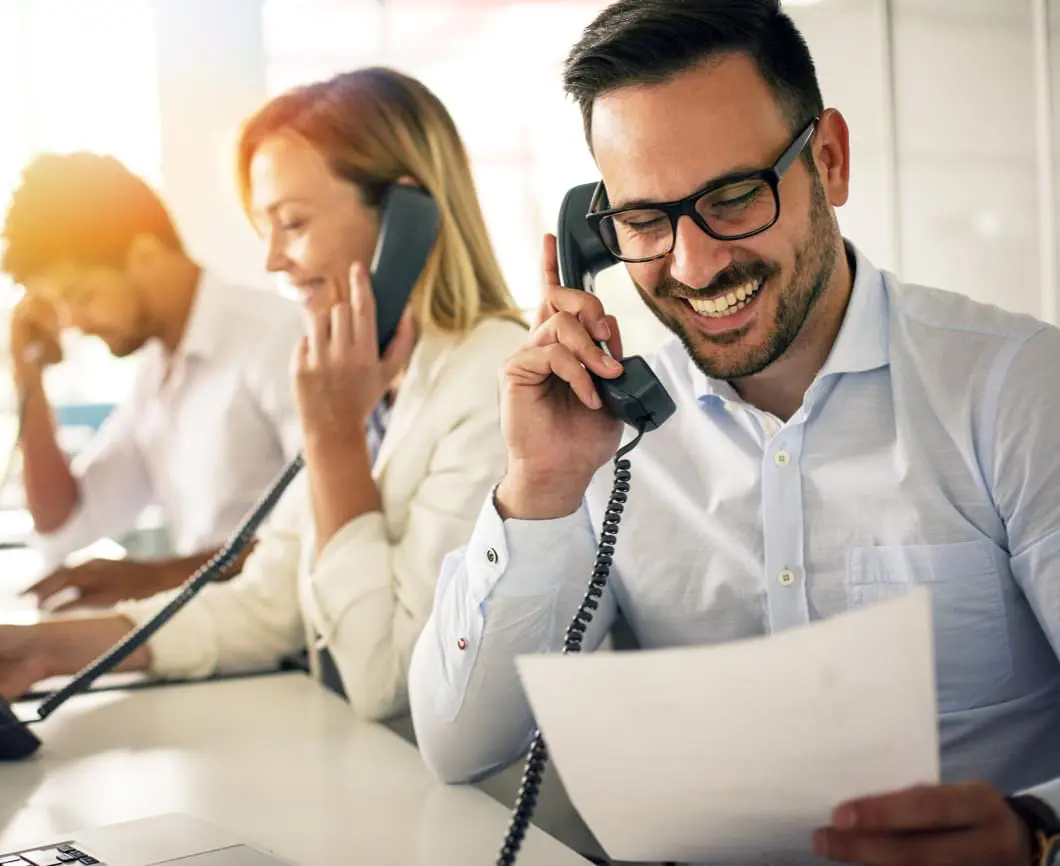