Ingredients for a successful data science team
With vast amounts of data now being generated by every business process and customer touch point, companies in almost every industry are focused on making the most of this data for competitive advantage. This has led to data science becoming a ‘must have’ capability, and data science teams being rapidly formed in businesses of all sizes, in all sectors.
Having spent over 20 years working in, setting up or running teams designed to leverage this competitive advantage, I wanted to share my observations on what it takes to build a great data science team. The starting point is a simple eight step framework that ensures you begin with the best foundation for success.
Step 1 – Recruit a diverse set of skills
We all know that the first step to building a team is to hire some people, but what skillsets are required to build a high-performing data science team? There is a lot of hype about unicorn data scientists who can do it all and emerging fields like Machine Learning (ML) Engineering and ML Ops but it’s not just about recruiting a bunch of bright technical experts; make sure you hire people who can understand your business, construct a problem statement and extract insights from science and analytics. These traits are as important as being able to use mathematical models to create the next algorithm.
Step 2 – Integrate into the business
Integrate your team into the business, as it is critical that data science delivers at the right part of the decision-making process. Successful teams are moving away from influencing decisions to augmenting or even automating decisions, this means science models must be incorporated in the business process. Therefore it is critical to invest time to understand these processes and build commercial understanding by being as close to the other departments as possible.
Step 3 – Tackle problems with an agile mindset
Analysts and data scientists don’t always like agile. They like logical flows, assembling all data before building features and before building models. But businesses often don’t have the luxury of time for this approach, wanting to see results quickly as they make day-to-day decisions. You need to strike a balance, and be flexible, responsive and adaptable by working in an agile way, adding more data and features as you progress, and building confidence early.
Step 4 – Drive accountability through measurement
It’s a tricky thing to do without results to benchmark, but you must estimate the value that your scientific models will add to the business if implemented. Even before you start, you should use your business knowledge to make assumptions about the outcomes. After implementation, measure the value and communicate this to stakeholders. We’re all impatient to move onto the next thing, but the best ammunition for arguing for more resource, budget or time is demonstrating the value that your data science team can deliver.
Step 5 – Influence by building trust
It’s natural that people will be sceptical of your team in the beginning, as many decisions are made on experience and gut instincts, not founded in data. Start small to build trust with your stakeholders, find those that are more open to the data-driven approach, and use your results in these areas to influence more widely.
Step 6 – Aim to take data science models to production
Preparing teams to productionise code helps you to move faster, this will mean you need engineering skills as well as modelling skills within the team but ensures that the business can see results from your work more rapidly.
Step 7 – Communicate your results as well as your existence
Make sure the business knows you are there, what your remit is, and how it will benefit the business overall. Communicate as widely and regularly as you can, demonstrating how and where data science is improving business processes, growing sales, helping win new customers, creating efficiencies.
Step 8 – Create a culture of learning
Data science is still evolving with new techniques, technologies and concepts being introduced frequently, rethink how you equip your team with the skills and knowledge they need. Bring the outside in, encourage curiosity, and learn from others, both within the business and the wider data science community.
Conclusion
With data scientist now feted as one of the most sought-after roles in business, and investment in building this capability being ramped up across the board, it can be confusing to know where to start but follow these principles and you’ll set your data science team up for the best possible success.
TOPICS
The latest insights from our experts around the world
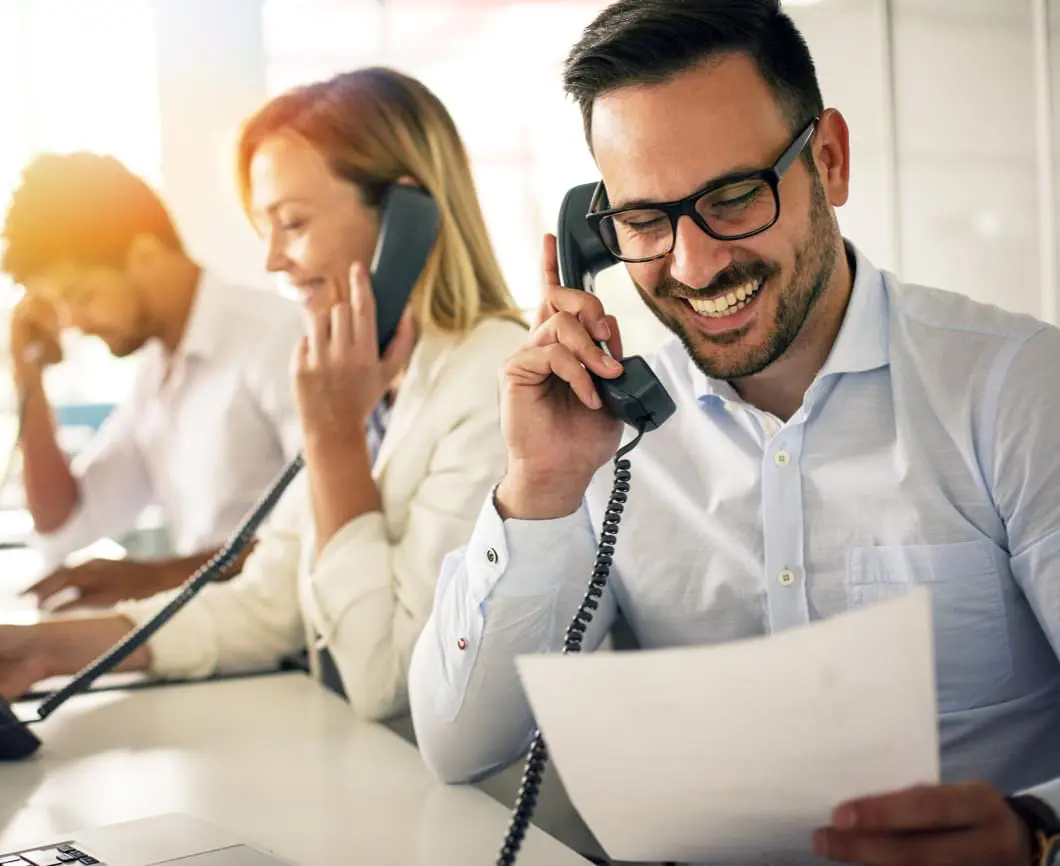