Personalisation, products, and price: some of the ways in which dunnhumby is using AI
Some ideas take a little longer than others to blossom. With ChatGPT celebrating its first birthday a few weeks back, it’s worth remembering that the “the first machine… capable of having an original idea”[1] actually dates back to 1958, and Frank Rosenblatt’s Perceptron. Nonetheless, there’s no doubt that the achievements of companies like OpenAI have helped to push artificial intelligence (AI) into mainstream conversation over the past 12 months.
With all the buzz around AI at the moment, one question that I increasingly find myself being asked is how exactly dunnhumby is using it. In truth, the answer is a little complex, because AI isn’t just one “thing”. It’s really an umbrella term, one that speaks to the idea that machines can be taught to perform all kinds of tasks normally associated with human intelligence[2]. And, under that umbrella, there are three key subsets of AI:
Machine Learning (ML)
ML is about taking data from the past and using it to train machines to carry out complex tasks without having to programme them to do so. A lot of our work involves ML, as I’ll explain in a moment.
Deep Learning (DL)
DL is where we move into the realm of neural networks, which try to mimic the human brain and the many layers within it. Some of the most common uses of DL include the analysis of images, videos, voice, and text.
Generative AI (GenAI)
Much of the current hype about AI revolves around GenAI, which is a very specific subset covering the creation of new content. As shown by the likes of ChatGPT and Midjourney, that could be new images, text, or even speech.
When we look at AI in this way, it doesn’t just take us into a much broader universe; it takes us much further back too. After all, when I joined dunnhumby in the late 1990s, we were already using machine learning to take a data-driven approach to solving problems. The really interesting thing is that the mathematical methods we use haven't really changed all that much during that time. The core principles remain the same – what’s different is the computing power we can apply to them and the amount of data available.
Twenty-five years ago, for instance, customer segmentations were already a core part of our capability. To create those, though, we would have relied on hours of manual analysis, picking out the individual variables we felt were likely to be the most predictive. Today, with a gigantic amount of computational power at our disposal, we can have a machine do that for us. Naturally, that quickly leads us into some very interesting areas.
Blending AI with deep industry knowledge
As noted above, out of those three subsets of AI, Machine Learning is the one we currently use the most. We do utilise Deep Learning to some extent, and we’re exploring Generative AI and how it could be applied to some of our existing models, but ML is where our focus lies today.
Across all those areas, though, we make a wide variety of tools and algorithms available to our experts. Each of those tools can be used to solve specific problems, and it’s our deep expertise in the grocery market that means we can pick the most appropriate tool for the task at hand – or, in other words, the one that will drive the best outcome.
As a whole, then, some form of AI is embedded into several of our propositions and products. That said, three areas in particular warrant specific mention.
1. Personalisation
Personalisation is about being able to understand every customer, every product, and every situation. That’s the core of what dunnhumby does, and our “recommendation engines” are fuelled by a range of AI and ML-based algorithms. Underpinning those is a relevancy science product called PAAR – Predictive Analytics Approach to Relevancy.
PAAR predicts what a customer is likely to buy next by analysing their prior purchasing behaviours. It does so by producing a relevancy score, one that denotes every customer’s propensity to buy “retention products” – items they’ve bought before. These scores can then be used across multiple different areas, stretching from product recommendations and coupons through to tailored content.
We also use ML algorithms for what is known as “predictive lifecycle science”, through which we predict a customer’s relationship with a retailer or brand over time.
2. Assortment
Assortment is fundamentally important to any retailer; if it’s not on the shelf, a shopper can’t buy it. That idea was integral to the development of dunnhumby Assortment, which draws on a wide range of AI and ML techniques in combination with our own proprietary algorithms. What results is a data science-led recommendation that is a true reflection of customer needs.
We use several different techniques within dunnhumby Assortment, including community detection (a way to explore clustering), unsupervised learning (via which algorithms learn patterns solely through unlabelled data), Natural Language Processing, ML, neural networks, and demand transfer. The latter of these focuses on the impact of introducing new products to a market.
3. Price optimisation
While ML is involved here, price optimisation is as much about the mathematical methods as it is the computing power. Nonetheless, by combining the two, we’re able to analyse vast amounts of data on pricing, competitors, customer behaviours, and more to identify the optimal price point for any given product. With different segmentations overlaid, that gives retailers the information that need to drive sales, increase margins, and do the right thing by their customers.
That latter point is particularly important. Certain customers are obviously more sensitive to price than others, and so we use unsupervised learning techniques to understand where that’s the case. That information can then be used to create the right prices for those customers where doing so matters most – key value lines, for instance.
In addition to the above – and the many other areas in which we’re using AI – we’re also exploring a range of other opportunities. Some of the most exciting include:
Automation and augmented intelligence
Looking back, we tended mainly to use information as way to back up existing hypotheses. If a buyer was thinking about introducing a new product into a range, for example, we could test the impact of doing so with data. Now, we’re moving to a place in which the algorithms can make those recommendations first. There’s also great potential in being able to automate low-complexity, high-frequency tasks.
Hyper-personalisation
The idea that we could personalise every single interaction with a customer isn’t new. In fact, dunnhumby can already do that – we create around 60bn personalised offers every year using ML. The main barrier is the ability to action that kind of personalisation at the individual level. Nonetheless, we recognise that customers expect personalised experiences, and we continue to advance our science, for example looking at techniques like graph neural networks.
Optimisation
Say a retailer has 3,000 items on promotion, and wants to put 100 of those in a huge display at the front of the store. Currently, it would take a high-end GPU about 44 years to test every combination, which is why you need business knowledge to constrain a problem and make it solvable. Quantum computing could provide a much bigger space in which to tackle those kinds of questions.
There are, of course, any number of other ways in which AI will end up being embedded in the work that we do, though what that looks like remains to be seen. What is clear, though, is that the ability to extract meaningful insights from vast amounts of information is now imperative for any business that wants to stay competitive. And, just as we have for so long already, we’ll be continuing to harness the power of AI to help our clients do just that.
[1] Race to AI: the origins of artificial intelligence, from Turing to ChatGPT – The Guardian, 28th October 2023
[2] This is one of the most commonly-accepted definitions of AI, though many others do also exist.
TOPICS
RELATED PRODUCTS
A look at dunnhumby’s unique Customer Data Science, which is at the core of everything we do.
dunnhumby ScienceEmbrace intelligent brand growth and increase sales
Maximise brand value and retailer collaborationSmarter operations and sustainable growth, powered by Customer Data Science
Perfect the science of shoppingThe latest insights from our experts around the world
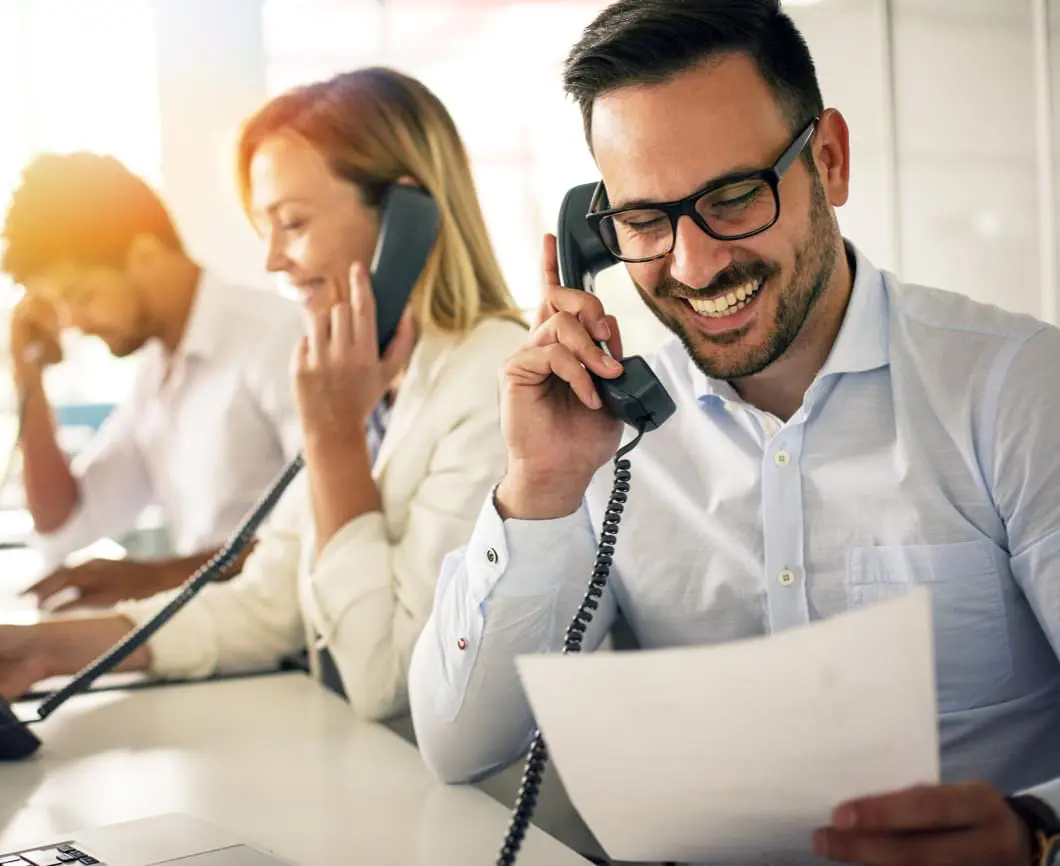