To prove the value of personalisation, we have to stay true to what’s right for the customer
When does personalisation become a bad thing? For those in the marketing, advertising, and – yes – data science industries, it can be a tricky question, one that comes without any easy answers. The tolerance threshold for tailored marketing communications can vary dramatically from person to person, after all, meaning that one shopper’s inspiration is another’s exasperation.
What we can say for sure, though, is that it’s never been easier for advertisers to stray into the wrong territory. FMCG retail in particular is an industry awash with data, ensuring that campaigns and ads can be targeted not just around pre-defined segments and assumed behaviours, but actual purchasing habits from individual – albeit anonymised – customers. Without the right controls, personalisation can quickly become unnecessarily intrusive.
Before we look at how to stop that from happening, I think it’s important that we acknowledge that not all forms of personalised advertising are the same. That’s particularly true in terms of the tactical ways in which personalisation can be used, but equally so at a much higher level too.
Relevant doesn’t always mean helpful
Take personalised – or what used to be known as “interest-based” – digital ads. I think we’re all very familiar with how these work; we use a search engine to look something up, or maybe discuss a topic in a social post or chat, and soon after that we begin to see ads featuring products relevant to that theme. Tents and rucksacks for nature enthusiasts, for example, or dog treats and toys for pet owners.
Those ads are obviously effective to some degree; not only are they still with us, a multi-billion dollar industry has been built around them. But thinking purely as consumers, I think we can rightly question how “useful” those adverts really are.
For a start, they can be fairly repetitive, with ads for the same item following us from site to site. They also tend to be disconnected from our actual behaviours, meaning that ads continue to appear even when we’ve purchased a featured item or one similar to it. And at their worst, these ads can feel quite intrusive – reminding us of the trail of data that we leave in our wake when we’re online, and thus ticking over into “creepy” territory.
I’d argue that things are very different when it comes to personalised advertising on ecommerce websites and apps, sites that we tend to visit specifically with a view to buying something. As such, there’s an element at play that doesn’t exist in the examples above: an opportunity for brands and retailers to use personalisation in a way that is genuinely helpful to customers.
Putting the customer first
Grocery retail is a prime example of the value of helpful personalisation. Shopping for groceries is a fairly utilitarian task, and one that few people truly relish – over say, planning a holiday. New products can inspire us, and there may be pockets of fun and excitement on a shopping trip, but we tend to primarily shop for food out of necessity rather than simply novelty. As a result, anything that can help us save time or make shopping easier tends to hold a great deal of value.
This is where something like dunnhumby’s own Recommenders product comes into its own. Recommenders deliver product suggestions to shoppers in real time. Those recommendations can take the form of everything from relevant complements and substitutions through to items that shoppers normally buy, but don’t have in their baskets when they’re about to check out.
Naturally, a huge amount of behind-the-scenes data science goes into those suggestions. To make sure that recommendations are accurate and effective, we need a complete, 360° view of the Customer, as well as predictive models that can accurately forecast their future needs. That’s something that we achieve through a combination of machine learning, artificial intelligence, and a great degree of computing power.
What results is a structure of around 15,000 datapoints for an average customer. Naturally these are all anonymised, but that still amounts to more than enough information to take us into territory that could easily be considered as intrusive or unhelpful. And that’s why it remains so critically important that we stay true to what’s right for the customer.
Integrity delivers results
To do that, we need to prioritise certain things. To ensure that recommendations are additive, not obstructive, we need to think about things like relevance, timeliness, and context. We – or the algorithms we create – need to really know that a customer is receiving suggestion for the right product in the right way and at the right time.
That’s crucial for brand advertisers, because it maximises the likelihood that a customer will add that item to their basket, and thus their return on advertising spend. But it’s equally – if not more – important for the retailer, since it has major implications for the quality of their customer experience. To prove that personalisation has long term value, we also need to ensure that it is something from which customers genuinely benefit.
More than anything, I think we’re on the cusp of a hugely exciting era for personalisation. There are a multitude of creative applications still waiting to be realised – from the automated delivery of frequently purchased items through to device-delivered reminders about product “use by” dates. The only limitation is our ability to think laterally about what data enables us to do.
Key to all of that, though, is an unwavering commitment to putting the customer at the heart of our decision making. So long as there’s a fair exchange – and that by sharing their data customers receive faster, simpler, and ultimately more valuable shopping experiences – then personalisation has a bright future ahead.
RELATED PRODUCTS
Amplify Customer understanding to create strategies that drive results
Customer First solutionsTurn Customer understanding into consistently engaging experiences
Customer Insights solutionsThe latest insights from our experts around the world
Disaggregating Forecasts
The six drivers of private brand innovation
AI's Regulatory Crossroads: Innovation vs. Control
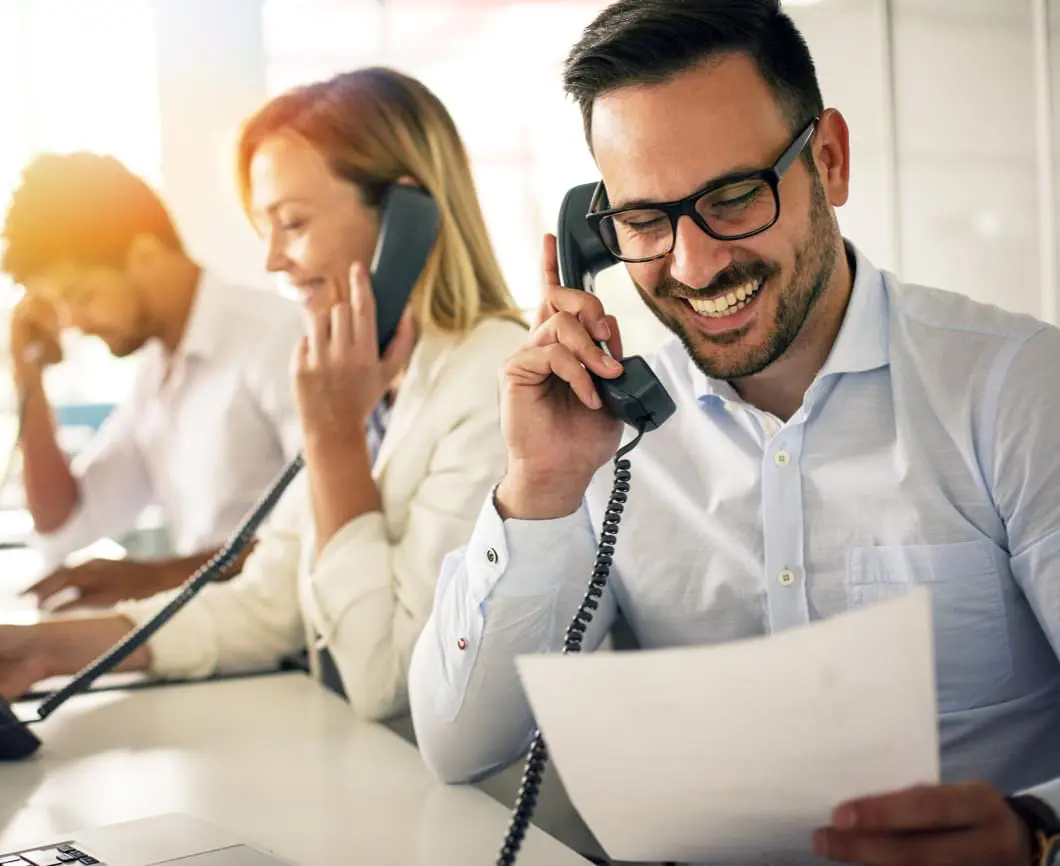
Speak to a member of our team for more information