Understanding the why: Causal AI and its value for retail
Causal Artificial Intelligence (AI), or causal inference, is the new and exciting science of cause and effect. It can enable businesses to understand and serve customers more effectively and efficiently than ever before, in a robust and transparent way. In this blog post series, dunnhumby Data Scientist Dimitra (Mimie) Liotsiou introduces this topic.
Cause and effect relationships are everywhere around us. When something happens – whether it’s a bottle smashing on the floor or a rocket launching into space – it’s because one or several other factors caused that event to take place.
In retail, cause and effect relationships are also everywhere. You’ve likely already thought about questions of cause and effect (causal questions), whether consciously or not, if you’ve ever wondered about questions such as:
- Why might a shopper put Brand A in their basket instead of Brand B?
- Why have a product’s sales been falling?
- What is the effectiveness of my investment in some initiative, proposition, or campaign?
Even though causal questions have been important to philosophers and scientists for centuries, obtaining a robust scientific understanding of causality has proved elusive.
However, there have recently been major scientific advances in this field. As Harvard professor Gary King put it: “More has been learned about causal inference in the last few decades than the sum total of everything that had been learned about it in all prior recorded history.”
We now have a scientific apparatus capable of taking us from mere statistical correlation to causation, by enabling us to disentangle and accurately measure the effects of different causes. In retail, this is key, so that data-driven insights will be more accurate and lead to the right course of action. And causal AI can do this at scale, for large, complex real-world problems, robustly and transparently.
From correlation to causation
Without the right approach, it becomes very easy to draw the wrong conclusions about cause and effect.
A well-known example is the following: a study found a correlation between chocolate consumption and the number of Nobel prizes won per capita per country. Does this mean eating more chocolate makes it more likely to win a Nobel? That doesn’t seem very plausible. Indeed, taking a step back to consider what else may be going on, further studies found that when adjusting for per capita income the correlation becomes insignificant. Income is a common cause of chocolate consumption and Nobel wins:
- High income per capita means people can afford treats like chocolate, and
- It also means a country generates more tax revenue, which can then be invested into education and research – increasing the likelihood of Nobel prize wins.
This chocolate-Nobels correlation is an example of a “spurious correlation” – a statistical relationship between two variables, that does not indicate a causal link from one variable to the other. Taking a broader look at the situation, we discover the correlation is explained by something else that’s going on, like a common cause (income, in this example).
Unfortunately, the data science, statistical, and machine learning methods commonly used today on Big Data can only tell us about correlations. They can’t tell us about causation, no matter how much data we collect, leaving us exposed to spurious correlations. This is where the causal inference approach comes in.
Causal AI for actionable retail insights
Retail data science insights need to be actionable. They need to give an accurate prediction of the effects caused by actions, so that, based on these insights, decision-makers can choose the right action to take next.
That means insights based on correlations will not suffice -- we don’t want a scenario where we invest in something based on a correlation, only to find it was spurious and the investment was misplaced or wasted.
Let’s consider another example: sending promotional coupons to existing customers of a product (i.e. customers with some degree of loyalty). Let’s say we find a strong positive relationship between receiving coupons and future purchases, so we might consider investing more in this coupon initiative. However, loyalty is a common cause of both receiving a coupon and of purchasing the product in the future regardless of whether a coupon was received. The result contains a spurious correlation, as the effects of the coupon and of prior loyalty are tangled together. Let’s say we adjust for loyalty, and the coupon’s effect turns out much weaker -- this initiative brings in little new revenue, as a lot of people would buy the product anyway due to pre-existing loyalty. So, investing more in this initiative may not be worthwhile after all.1
More generally, questions requiring actionable predictive insights about effects of actions are ubiquitous in retail, and very important. Further examples include questions about, for example, the impact of a media campaign, range change, price change, or a rewards programme, on key outcomes like customer spend, in-store footfall, or loyalty. Broader questions like “which factors influence average customer spend?”, or questions about uplift or incrementality, also fall in this category.
Irrespective of what you want to know, to answer a causal question accurately, you need to use causal methods.
Reality is complex: Accounting for multiple causal factors
The Nobel and coupon examples were simple illustrations of the causal inference approach. More generally, to go beyond correlations, we need to consider the wider picture of the various underlying causal factors and relationships at play in the real world, and appropriately account for these.
If we wanted to understand the impact of advertising a product on its total sales, for example, there is a whole range of variables that we could adjust for, such as variables capturing characteristics of:
- The product itself, e.g. price, quality
- The category the product sits in
- Competing products
- Competing retailers
- Time of year and seasonal events
- The state of the economy
- Consumer preferences and behaviour
Clearly, there are many factors to consider here. Should we adjust for all of them? Just some? If so, which ones should we include? Which should we leave out?
This is a critical and complex question, as indiscriminately adjusting for all, or as many as possible, variables can also introduce spurious correlations (bias) into results. Fortunately, causal AI provides the solution, as it can automatically determine the right set of variables to adjust for in a given context.
Reaping the benefits of causal AI entails moving from the traditional data science pipeline, which hinges entirely on correlations, to the causal pipeline, in which traditional data science methods still feature but are only one stage of the process.
Harnessing these advanced causal methods comes with its own nuances and complexities, so expertise and care is needed to apply them effectively.
At dunnhumby, we are actively working on bringing causal AI to bear on a wide range of retail problems.
A more in-depth look at how causal AI works, and at the causal pipeline, is something I’ll cover in the second post in this series, coming soon. In the meantime, to discover more, please see this presentation I gave at the recent London Data Science Festival.
[1] A similar example, with targeted online adverts instead of coupons, is discussed here
TOPICS
RELATED PRODUCTS
Truly understand your Customers and unlock your Customer First transformation with Strategy Development, Research & Insights and Organisation Engagement
Design the right strategy with the Customer at the centerA look at dunnhumby’s unique Customer Data Science, which is at the core of everything we do.
Combining the latest techniques, algorithms, processes and applicationsThe latest insights from our experts around the world
AI's Regulatory Crossroads: Innovation vs. Control
Why you need a demand model
AI: three breakout applications for consumer brands
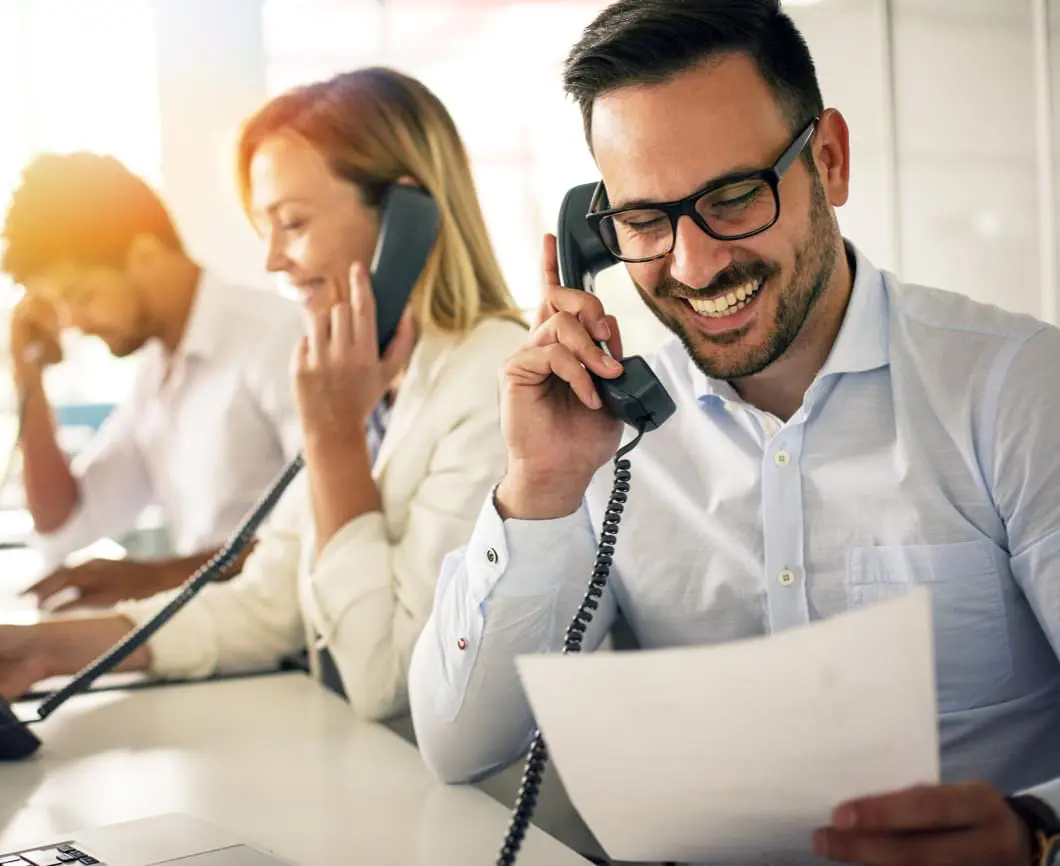
Speak to a member of our team for more information